包阅导读总结
1. 关键词:Llama 3、Meta、Open Source、Data、Inference
2. 总结:Meta 的 Llama 3 是其目前最强大的公开可用 LLM,Llama 3.1 带来新工作流程。在 AI Infra @ Scale 2024 上,Meta 工程师探讨了从数据、训练到推理构建和实现 Llama 3 的每一步,包括开放源 AI 愿景、数据相关事项、大规模训练以及推理处理。
3. 主要内容:
– Llama 3 是 Meta 最强大的公开可用 LLM,Llama 3.1 具备新优势。
– 在 AI Infra @ Scale 2024 上展开讨论。
– 产品总监 Joe Spisak 谈 Llama 历史和 Meta 开源 AI 愿景。
– 软件工程师 Delia David 讨论 GenAI 的数据相关内容,包括多样性、数量和新鲜度,及数据提取与准备。
– 软件工程师 Kaushik Veeraraghavan 讲述 Meta 如何大规模训练 Llama 及相关投入。
– 生产工程师 Ye (Charlotte) Qi 探讨 Meta 对 Llama 的推理处理,包括优化、缩放和面临的挑战。
思维导图:
文章地址:https://engineering.fb.com/2024/08/21/production-engineering/bringing-llama-3-to-life/
文章来源:engineering.fb.com
作者:Engineering at Meta
发布时间:2024/8/26 23:38
语言:英文
总字数:239字
预计阅读时间:1分钟
评分:92分
标签:Llama 3,大型语言模型,Meta,开源 AI,AI 基础设施
以下为原文内容
本内容来源于用户推荐转载,旨在分享知识与观点,如有侵权请联系删除 联系邮箱 media@ilingban.com
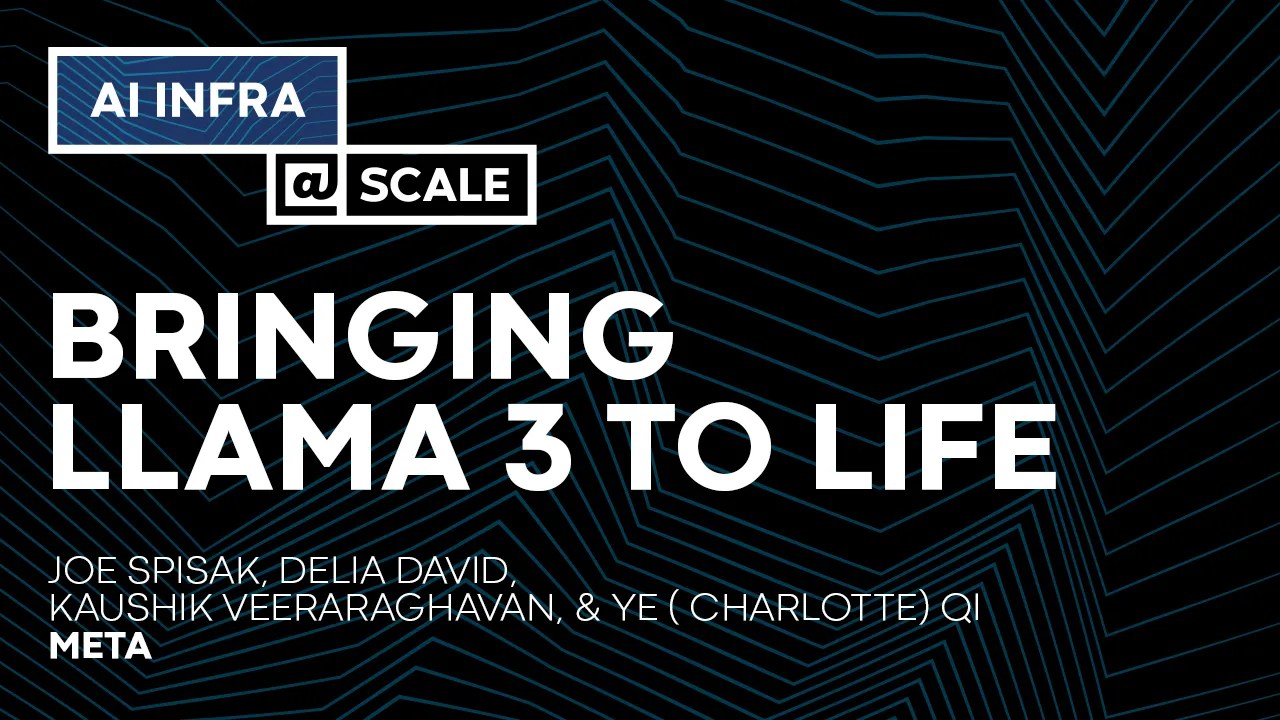
Llama 3 is Meta’s most capable openly-available LLM to date and the recently-released Llama 3.1 will enable new workflows, such as synthetic data generation and model distillation with unmatched flexibility, control, and state-of-the-art capabilities that rival the best closed source models.
At AI Infra @ Scale 2024, Meta engineers discussed every step of how we built and brought Llama 3 to life, from data and training to inference.
Joe Spisak, Product Director and Head of Generative AI Open Source at Meta, talks about the history of Llama and Meta’s overarching vision for open source AI.
He’s joined by Delia David, a software engineer at Meta, to discuss all things data-related for GenAI. David covers the diversity, volume, and freshness of data needed for GenAI and how different data types should be extracted and prepared.
Kaushik Veeraraghavan, a software engineer at Meta, discusses how Meta trains Llama at scale and delves into the data center, networking, and software investments that have enabled the development of Meta’s Llama 3 models.
Finally, Ye (Charlotte) Qi, a production engineer at Meta, discusses how Meta handles inference for Llama. Optimizing and scaling LLM inference is important for enabling large-scale product applications. Qi introduces key parallelism techniques that help scale model sizes and context windows, which in turn influence inference system designs. She also discusses practical challenges associated with deploying these complex serving paradigms throughout Meta’s internal cloud to our data center of heterogeneous hardware.
VIDEO