包阅导读总结
1. 关键词:Long Code Arena、AI 模型、软件项目、基准测试、代码开发
2. 总结:本文介绍了 JetBrains 推出的 Long Code Arena 基准测试集,它要求 AI 模型以整个项目为输入,涵盖多种任务。测试结果显示当前模型仍有改进空间,希望该数据集能推动代码模型研究和软件工具改进。
3. 主要内容:
– Long Code Arena 介绍
– AI 在软件开发各方面的应用及背景。
– 强调上下文规模对模型输出的影响,软件工程中最新模型能处理整个项目。
– 基准测试任务
– 测试模型利用给定库完成任务的能力,GPT-4 表现最佳但仍有提升空间。
– 评估模型修复失败 CI 构建的能力,结果显示实用效果不佳。
– 考察模型的代码完成能力,按不同类别测试,通过组合上下文有改进。
– 测试生成提交消息,复杂数据表现差,发布数据集供未来研究。
– 测试模型的错误定位能力,GPT-4 表现较好。
– 测试模型理解并总结整个项目的能力,GPT-4 概率优于其他模型。
– 未来展望
– Long Code Arena 是迈向更好代码模型的重要一步。
– 计划用于改进 JetBrains 工具,推动领域发展。
思维导图:
文章来源:blog.jetbrains.com
作者:Yaroslav Golubev
发布时间:2024/8/19 11:45
语言:英文
总字数:1214字
预计阅读时间:5分钟
评分:86分
标签:智能软件工程,长代码测试场,AI 基准测试,代码生成,CI 构建修补
以下为原文内容
本内容来源于用户推荐转载,旨在分享知识与观点,如有侵权请联系删除 联系邮箱 media@ilingban.com
Long Code Arena: How Well Can AI Models Understand Your Entire Project?
In the last couple of years, AI has found its way into virtually all aspects of software development. We know that AI models are getting smarter, and we can clearly see how they have become better at seemingly everything, but why is this happening? One factor that is changing is context size, which is how much information models are able to receive as input. Basically, as the models become capable of processing larger amounts of data, they can provide better outputs.
In software engineering specifically, the most recent models have context sizes that allow them to take entire projects – tens or even hundreds of files! – as input, and this impacts a variety of software development tasks. However, with the rapid advancements in the field, the benchmarks are lagging behind. Most researchers still evaluate the newest code models at the scale of files or often even just individual functions.
But no more!
Recently, JetBrains Research introduced Long Code Arena – a set of six benchmarks that require the models to take an entire project as input. All the tasks in the suite contain high-quality reference solutions, manually collected and verified. In this blog post, we will share with you what these benchmarks are and how they will help researchers all over the world to train the next generation of smart AI models for code. If you’d like to learn about Long Code Arena in more detail, check out this preprint of the formal paper, which has received a lot of positive feedback.
Code generation is one of the most common uses of AI for coding. However, one aspect of this use case that can be difficult to control is the exact kind of code the model will generate for you. If you just ask it to complete a task, it might introduce new dependencies that will make your project harder to maintain or it may awkwardly use built-in solutions when more convenient libraries exist.
Our first benchmark tests a smarter way to approach this scenario. As usual, the model receives a task that it must complete, but it also receives the contents of a given library, and it must complete the task while making heavy use of the library’s APIs. This tests the model’s ability to process a full library – both code and documentation – and provide results that rely on what you want. We found that GPT-4 performs the best, but even it only uses 37% of the APIs found in the reference solutions, leaving huge room for further improvement.
Next up, we test the models on their ability to fix a failing CI build. We give the model an entire repository at the moment of failure and the logs of the failed run, and the model has to generate a patch. For this task, we developed an interactive evaluation system! The patch that the model generates gets applied, and then the fixed version of the build is run live in GitHub Actions. This represents an evaluation pipeline that’s as close to real-world conditions as possible.
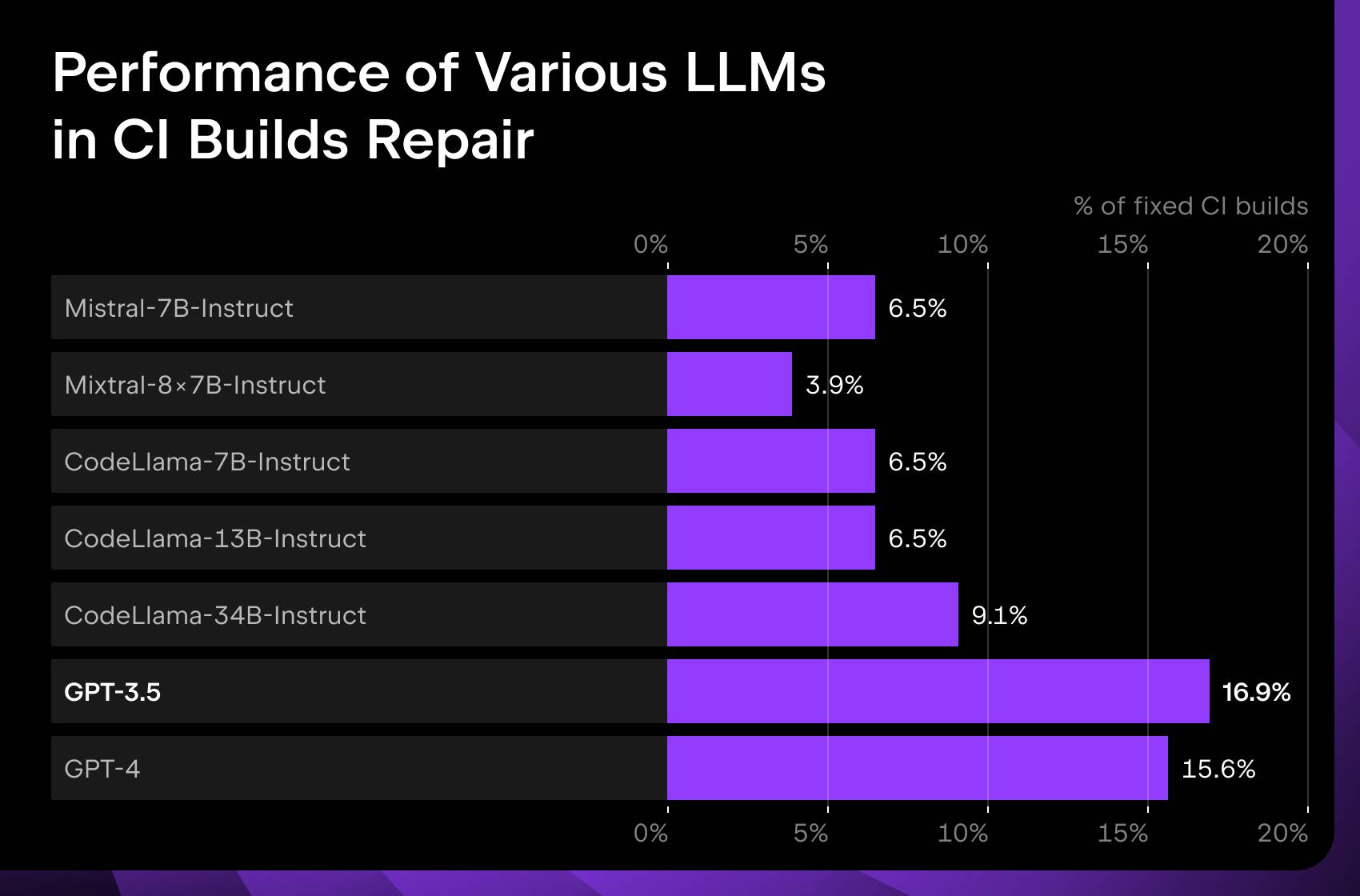
The results show that even the best model – GPT-3.5 in this case – can only fix 17% of failing builds, indicating just how much work yet needs to be done for AI to be practically useful in this context.
Together with code generation, code completion is among the most popular uses of AI for coding, and so of course we want to evaluate it. The model receives the full project and the contents of the file, and it then has to generate the next line in this file. The model needs to use the entire project because some of the lines that need to be completed contain functions or classes from other files in the repository. To test this exhaustively, our benchmark includes a detailed categorization of lines by the different functions and classes that they contain, and thus in a way, by difficulty.
Unsurprisingly, our results show that it is harder for the models to correctly generate a line that contains functions from other parts of the project. However, we tested a way to compose context using files that are closest in the file tree to the completion file, and this resulted in a noticeable improvement.
The generation of commit messages is an established task in software engineering research, and it is highly useful in practice. Models take in the code diff and outputs a description of it in natural language. While this does not require the full project, existing benchmarks still only evaluate the models on highly filtered, pristine diffs and commit messages, whereas reality can be much more complicated. To test a more complex case, we collected a dataset of large commits, ones with hundreds of lines of code and thousands of symbols.
Again, unsurprisingly, the models perform worse on such data than on smaller, more atomic commits. If we want our future models to be able to handle even larger amounts of data, our dataset will come in handy. For instance, we released sources of all repositories appearing in our dataset, allowing future researchers to explore whether the context of the entire project might be beneficial for this task as well.
Bug localization tests the ability of models to understand a repository, with all its nuances and complexities. Given the full repository and an issue that contains a bug description, the model must point out which files need to be fixed to solve the issue. Here, too, GPT-4 performed better than the other models.
Finally, the last task evaluates the ability of the model to understand the full project even more explicitly. Summarization is another key way AI models work with code. However, it also is usually tested by writing comments or docstrings for individual functions, while modern models can do so much more. In our benchmark, we give the model a full module or repository and a short instruction about what type of documentation is needed, for example, a README. The model then has to process and summarize it all.
This task also features a novel metric. While there are a lot of metrics for comparing texts, they were developed for short strings and might not work on entire pages of documents. For that reason, we employ AI models to evaluate other AI models: We give an LLM the relevant code and two versions of documentation – the reference and the generated one – and ask it to identify which version better matches the input code. We do this several times with the order randomized to account for potential biases.
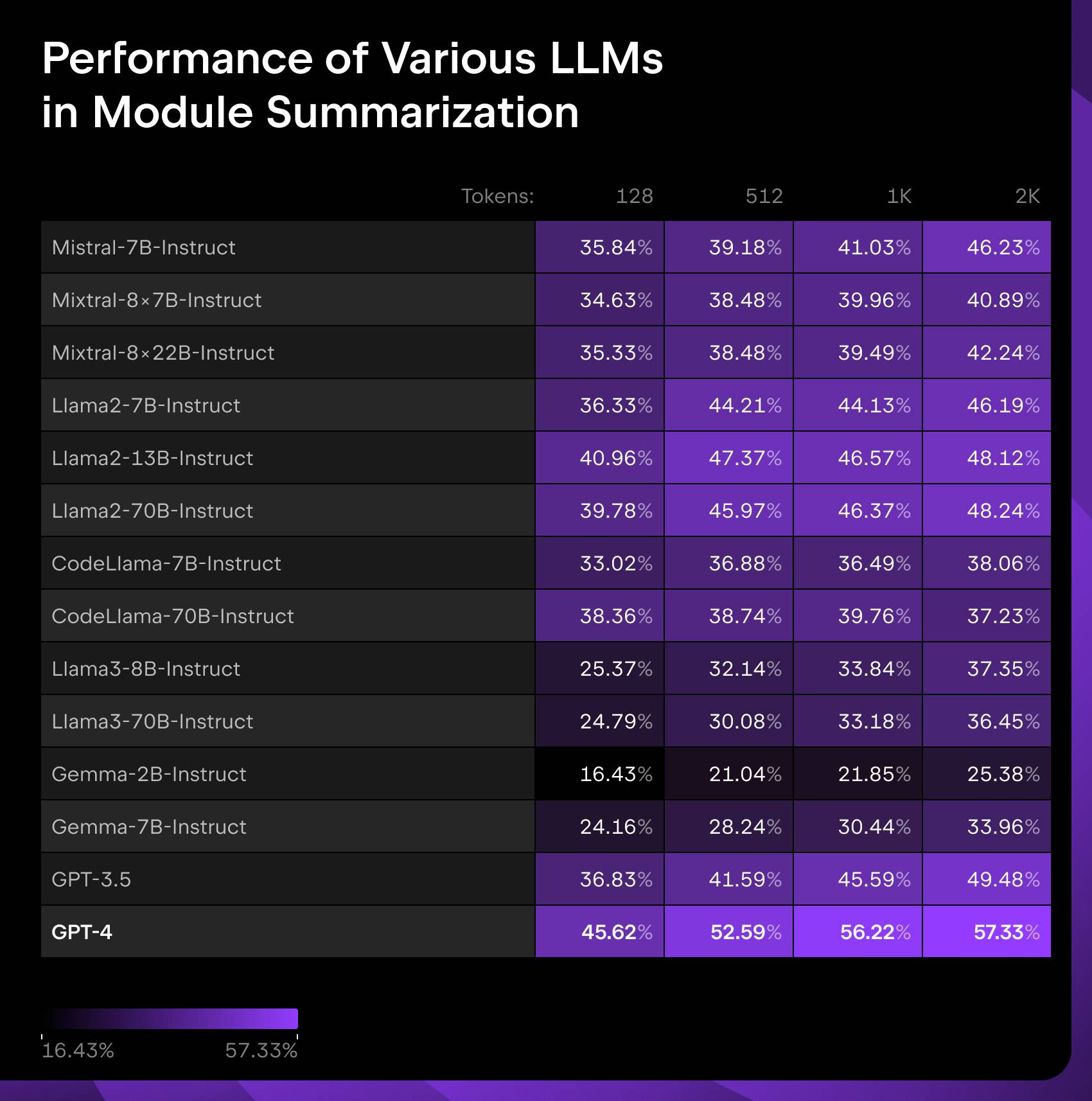
Testing different models on different context sizes, we can see that GPT-4 beats other models here as well, demonstrating a 45–57% probability of being better than the reference.
Looking forward
Long Code Arena represents an important step towards better code models, and it is the first dataset with large contexts in the field. We hope that it will be useful for the research community. We already plan to use it to improve the models in JetBrains tools, and with it we will continue to move the field forward!