包阅导读总结
1. 关键词:多代理、客服系统、任务、交互、流程
2. 总结:本文介绍了构建多代理客服系统的原因,详细描述了所建系统的构成、流程和实际运行情况,并展示了相关代码核心部分,包括不同代理的功能和交互方式。
3. 主要内容:
– 为何构建
– 大规模聊天机器人需求,单个提示复杂,设计多代理系统。
– 构建内容
– 四个基本“任务”代理:股票查询、认证、账户余额、转账。
– 三个“元”代理:礼宾、协调、延续。
– 全局状态跟踪用户和当前状态。
– 系统运行
– 以转账为例展示流程,包括与各代理交互、检查认证和余额等。
– 代码
– 核心是中央循环,通过协调代理决定下一个发言者。
– 展示协调代理严格提示相关代码。
思维导图:
文章地址:https://www.llamaindex.ai/blog/building-a-multi-agent-concierge-system
文章来源:llamaindex.ai
作者:LlamaIndex
发布时间:2024/7/17 0:00
语言:英文
总字数:2337字
预计阅读时间:10分钟
评分:93分
标签:多代理系统,聊天机器人,LlamaIndex,任务编排,代理链
以下为原文内容
本内容来源于用户推荐转载,旨在分享知识与观点,如有侵权请联系删除 联系邮箱 media@ilingban.com
Why build this?
Interactive chat bots are by this point a familiar solution to customer service, and agents are a frequent component of chat bot implementations. They provide memory, introspection, tool use and other features necessary for a competent bot.
We have become interested in larger-scale chatbots: ones that can complete dozens of tasks, some of which have dependencies on each other, using hundreds of tools. What would that agent look like? It would have an enormous system prompt and a huge number of tools to choose from, which can be confusing for an agent.
Imagine a bank implementing a system that can:
- Look up the price of a specific stock
- Authenticate a user
- Check your account balance
- Which requires the user be authenticated
- Transfer money between accounts
- Which requires the user be authenticated
- And also that the user checks their account balance first
Each of these top-level tasks has sub-tasks, for instance:
- The stock price lookup might need to look up the stock symbol first
- The user authentication would need to gather a username and a password
- The account balance would need to know which of the user’s accounts to check
Coming up with a single primary prompt for all of these tasks and sub-tasks would be very complex. So instead, we designed a multi-agent system with agents responsible for each top-level task, plus a “concierge” agent that can direct the user to the correct agent.
What we built
We built a system of agents to complete the above tasks. It’s open-source! There are four basic “task” agents:
- A stock lookup agent (which takes care of sub-tasks like looking up symbols)
- An authentication agent (which asks for username and password)
- An account balance agent (which takes care of sub-tasks like selecting an account)
- A money transfer agent (which takes care of tasks like asking what account to transfer to, and how much)
There are also three “meta” agents:
- A concierge agent: this agent is responsible for interacting with the user when they first arrive, letting them know what sort of tasks are available, and providing feedback when tasks are complete.
- An orchestration agent: this agent never provides output directly to the user. Instead, it looks at what the user is currently trying to accomplish, and responds with the plain-text name of the agent that should handle the task. The code then routes to that agent.
- A continuation agent: it’s sometimes necessary to chain agents together to complete a task. For instance, to check your account balance, you need to be authenticated first. The authentication agent doesn’t know if you were simply trying to authenticate yourself or if it’s part of a chain, and it doesn’t need to. When the authentication agent completes, the continuation agent checks chat history to see what the original task was, and if there’s more to do, it formulates a new request to the orchestration agent to get you there without further user input.
A global state keeps track of the user and their current state, shared between all the agents.
The flow of the the system looks something like this:
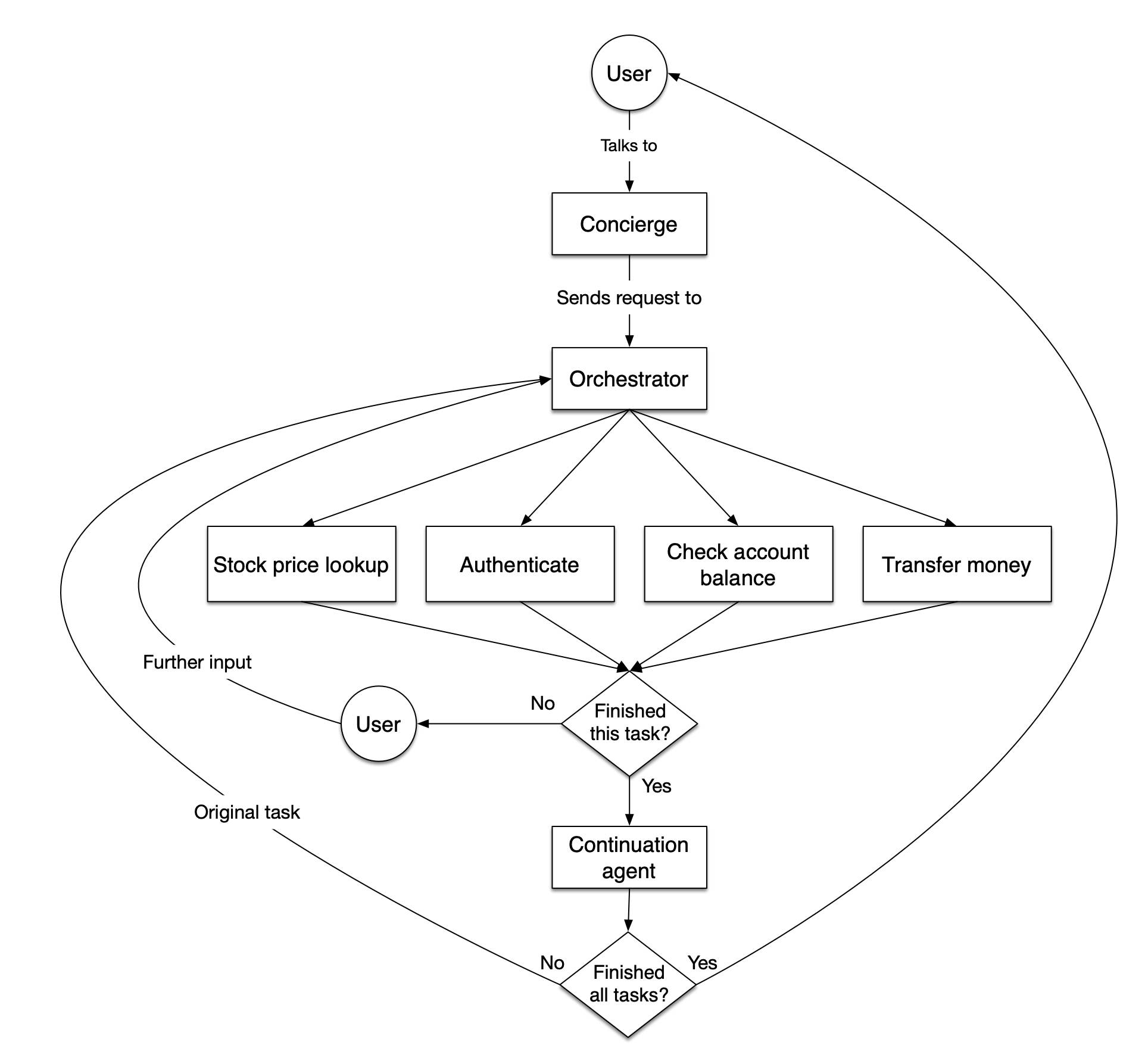
The system in action
To get a sense of how this works in practice, here’s sample output including helpful debug statements. Output that would be ordinarily shown to the user has two >>
, while user input has one >
.
At the beginning of the conversation nothing’s happened yet, so you get routed to the concierge:
No current speaker, asking orchestration agent to decideConcierge agent selected>> Hi there! How can I assist you today? Here are some things I can help you with:>> Looking up a stock price>> Authenticating you>> Checking an account balance (requires authentication first)>> Transferring money between accounts (requires authentication and checking an account balance first)>> What would you like to do?> Transfer money
The “transfer money” task requires authentication. The orchestration agent checks if you’re authenticated while deciding how to route you (it does this twice for some reason, it’s a demo!):
No current speaker, asking orchestration agent to decideOrchestrator is checking if authenticatedOrchestrator is checking if authenticatedAuth agent selected
It correctly determines you’re not authenticated, so it routes you to the authentication agent:
>> To transfer money, I need to authenticate you first. Could you please provide your username and password?> seldo
This is a fun part: you’ve provided input, but it’s not sufficient to complete the task (you didn’t give a password). So when the flow goes back to the orchestration agent, the global state indicates that the “authenticate” agent is already running and hasn’t completed yet, so it routes back to the authentication agent, and does that again for the password:
There's already a speaker: authenticateAuth agent selectedRecording username>> Thank you! Now, could you please provide your password?> monkeyThere's already a speaker: authenticateAuth agent selectedLogging in seldoChecking if authenticatedAuthentication is complete
Now the auth agent has called a done() function that indicates to the global state that it has completed its task. So the flow now goes to the continuation agent, which looks at the chat history and sees that the user was trying to transfer money. So it generates a prompt, as if spoken by the user, and sends that to the orchestration agent:
>> You have been successfully authenticated. Another agent will assist you with transferring money.Asking the continuation agent to decide what to do nextContinuation agent said "I would like to transfer money."No current speaker, asking orchestration agent to decideOrchestrator checking if account has a balanceOrchestrator checking if account has a balanceAccount balance agent selected
Now you’re authenticated, but you haven’t checked your balance yet, which the orchestration agent knows is necessary for transferring money. So it routes you to the account balance agent (after checking twice for some reason):
>> Before you can transfer money, you need to check your account balance. Let's start by looking up your account balance. Could you please provide the name of the account you're interested in?> CheckingThere's already a speaker: account_balanceAccount balance agent selectedLooking up account ID for CheckingLooking up account balance for 1234567890Account balance lookup is complete>> Your Checking account has a balance of $1000. Another agent will assist you with transferring money.Asking the continuation agent to decide what to do nextContinuation agent said "I would like to transfer money."No current speaker, asking orchestration agent to decideTransfer money agent selected
The account balance agent asks you which account, uses a tool to get the ID for that account, and then marks itself as done. The continuation agent kicks in again and sees that you still haven’t completed your original task of transferring money, so it prompts the orchestrator agent again. Unfortunately the orchestrator gets a little confused, and loops twice before finally routing you to the transfer money agent:
Money transfer is complete>> Another agent will assist you with transferring money.Asking the continuation agent to decide what to do nextContinuation agent said "I would like to transfer money."No current speaker, asking orchestration agent to decideTransfer money agent selectedMoney transfer is complete>> Another agent will assist you with transferring money.Asking the continuation agent to decide what to do nextContinuation agent said "I would like to transfer money."No current speaker, asking orchestration agent to decideOrchestrator checking if account has a balanceTransfer money agent selected>> You have already checked your account balance. Please provide the following details to proceed with the money transfer:>> 1. The account ID to which you want to transfer the money.>> 2. The amount you want to transfer.> To account ID 1234324There's already a speaker: transfer_moneyTransfer money agent selected>> How much would you like to transfer to account ID 1234324?> 500There's already a speaker: transfer_moneyTransfer money agent selectedChecking if balance is sufficientTransferring 500 from 1234567890 account 1234324Money transfer is complete>> The transfer of $500 to account ID 1234324 has been successfully completed. If you need any further assistance, feel free to ask!Asking the continuation agent to decide what to do nextContinuation agent said no_further_tasks
We’ve reached the end of the task! The continuation agent sees that there are no further tasks, and routes you back to the concierge.
The code
Now let’s look at some highlights of the code that gets all of this done. The core of the system is a central loop that runs forever. At the core of that is a very simple block that simply asks the orchestration agent who should speak next, and sets the next_speaker
value which is contained in the state object that is passed between all the agents. Note that if there’s already a sub-agent speaking, that agent gets to keep speaking.
current_history = root_memory.get()if (state["current_speaker"]): print(f"There's already a speaker: {state['current_speaker']}") next_speaker = state["current_speaker"]else: print("No current speaker, asking orchestration agent to decide") orchestration_response = orchestration_agent_factory(state).chat( user_msg_str, chat_history=current_history ) next_speaker = str(orchestration_response).strip()
The orchestration agent has a very strict prompt; its output only goes to other machines. It includes a natural-language summary of the dependencies between agents:
system_prompt = (f""" You are on orchestration agent. Your job is to decide which agent to run based on the current state of the user and what they've asked to do. Agents are identified by short strings. What you do is return the name of the agent to run next. You do not do anything else. The current state of the user is: {pprint.pformat(state, indent=4)} If a current_speaker is already selected in the state, simply output that value. If there is no current_speaker value, look at the chat history and the current state and you MUST return one of these strings identifying an agent to run: * "{Speaker.STOCK_LOOKUP.value}" - if they user wants to look up a stock price (does not require authentication) * "{Speaker.AUTHENTICATE.value}" - if the user needs to authenticate * "{Speaker.ACCOUNT_BALANCE.value}" - if the user wants to look up an account balance * If they want to look up an account balance, but they haven't authenticated yet, return "{Speaker.AUTHENTICATE.value}" instead * "{Speaker.TRANSFER_MONEY.value}" - if the user wants to transfer money between accounts (requires authentication and checking an account balance first) * If they want to transfer money, but is_authenticated returns false, return "{Speaker.AUTHENTICATE.value}" instead * If they want to transfer money, but has_balance returns false, return "{Speaker.ACCOUNT_BALANCE.value}" instead * "{Speaker.CONCIERGE.value}" - if the user wants to do something else, or hasn't said what they want to do, or you can't figure out what they want to do. Choose this by default. Output one of these strings and ONLY these strings, without quotes. NEVER respond with anything other than one of the above five strings. DO NOT be helpful or conversational. """)
A simple if-else block takes the output of the orchestration agent and uses it to instantiate the sub-agent to run next. This is when the state object gets passed to each sub-agent:
if next_speaker == Speaker.STOCK_LOOKUP: print("Stock lookup agent selected") current_speaker = stock_lookup_agent_factory(state) state["current_speaker"] = next_speaker elif next_speaker == Speaker.AUTHENTICATE: print("Auth agent selected") current_speaker = auth_agent_factory(state) state["current_speaker"] = next_speaker elif next_speaker == Speaker.ACCOUNT_BALANCE: print("Account balance agent selected") current_speaker = account_balance_agent_factory(state) state["current_speaker"] = next_speaker elif next_speaker == Speaker.TRANSFER_MONEY: print("Transfer money agent selected") current_speaker = transfer_money_agent_factory(state) state["current_speaker"] = next_speaker elif next_speaker == Speaker.CONCIERGE: print("Concierge agent selected") current_speaker = concierge_agent_factory(state) else: print("Orchestration agent failed to return a valid speaker; ask it to try again") is_retry = True continue
And then the full chat history is passed as part of a regular chat message to the newly-instantiated agent:
response = current_speaker.chat(user_msg_str, chat_history=current_history)
The agent reads its prompt and the user input and decides what to say. As we saw in our very first block of code, if the speaker is already selected, then the loop will keep talking to the current sub-agent. This continues until the sub-agent has completed its task, at which point its prompt instructs it to call the done()
function:
def done() -> None: """When you complete your task, call this tool.""" print("Money transfer is complete") state["current_speaker"] = None state["just_finished"] = True
This modifies the state, setting the current speaker to none. This triggers the outer loop to run the continuation agent, to see if there’s anything else to do:
elif state["just_finished"] == True: print("Asking the continuation agent to decide what to do next") user_msg_str = str(continuation_agent_factory(state).chat(""" Look at the chat history to date and figure out what the user was originally trying to do. They might have had to do some sub-tasks to complete that task, but what we want is the original thing they started out trying to do. Formulate a sentence as if written by the user that asks to continue that task. If it seems like the user really completed their task, output "no_further_task" only. """, chat_history=current_history)) print(f"Continuation agent said {user_msg_str}") if user_msg_str == "no_further_task": user_msg_str = input(">> ").strip() state["just_finished"] = False
The continuation agent’s prompt instructs it to reply as if it were the user asking to perform a task, or to output no_further_task
if there’s no more to do. If there’s a new task, the output of the continuation agent becomes the input to the orchestrator, which selects a new speaker. If there’s no further task, the loop pauses for more user input.
And that’s the full system! The sub-agents can be arbitrarily complicated, multi-turn systems in themselves, and the outer loop doesn’t need to know how they work, just how they depend on each other.
What’s next
We think there’s some novel stuff in here: coordinating multiple agents “speaking” simultaneously, creating implicit “chains” of agents through natural language instructions, using a “continuation” agent to manage those chains, and using a global state this way. We’re excited to see what you do with the patterns we’ve laid out here. Don’t forget to check out the open-source repo!