包阅导读总结
1. 关键词:Retrieval-Augmented Generation、LLM、Knowledge Base、Search、Improvement
2. 总结:文本介绍了检索增强生成(RAG)的基本概念,包括其是预训练 LLM 与外部知识库的结合,还给出简单实现方法。接着提出五条改进 RAG 管道的途径,如混合搜索、数据清理、提示工程、评估、数据收集。最后提到未来可基于评估套件测试更多改进方法。
3. 主要内容:
– RAG 简介:
– 是预训练 LLM 与外部可搜索知识库的组合
– 推理时在知识库中搜索相关文本并添加到 LLM 的提示中
– 简单实现 RAG:
– 分离知识库为固定大小的块
– 用嵌入模型向量化每个块
– 推理时向量化输入/查询并通过向量搜索找到相关块
– 将相关块添加到 LLM 的提示中
– 改进 RAG 管道的途径:
– 混合搜索:采用词汇和向量检索结合及重排序
– 数据清理:预处理或清洁数据,标准化、过滤和提取
– 提示工程:制作包含相关数据并能引导 LLM 产生可靠输出的提示
– 评估:建立可重复准确的评估管道,分别评估各组件
– 数据收集:收集数据用于改进应用
– 未来展望:基于评估套件测试更多改进方法
思维导图:
文章地址:https://stackoverflow.blog/2024/08/15/practical-tips-for-retrieval-augmented-generation-rag/
文章来源:stackoverflow.blog
作者:Cameron R. Wolfe,PhD
发布时间:2024/8/15 16:00
语言:英文
总字数:534字
预计阅读时间:3分钟
评分:90分
标签:RAG,检索增强生成,提示词工程,数据清洗,评估
以下为原文内容
本内容来源于用户推荐转载,旨在分享知识与观点,如有侵权请联系删除 联系邮箱 media@ilingban.com
At the highest level, RAG is a combination of a pretrained LLM with an external (searchable) knowledge base. At inference time, we can search for relevant textual context within this knowledge base and add it to the LLM’s prompt. Then, the LLM can use its in context learning abilities to leverage this added context and produce a more factual/grounded output.
Simple implementation: We can create a minimal RAG pipeline using a pretrained embedding model and LLM by:
1. Separating the knowledge base into fixed-size chunks.
2. Vectorizing each chunk with an embedding model.
3. Vectorizing the input/query at inference time and using vector search to find relevant chunks.
4. Adding relevant chunks into the LLM’s prompt.
This simple approach works, but building a high-performing RAG application requires much more. Here are five avenues we can follow to refine our RAG pipeline.
(1) Hybrid search: At the end of the day, the retrieval component of RAG is just a search engine. So, we can drastically improve retrieval by using ideas from search. For example, we can perform both lexical and vector retrieval (i.e., hybrid retrieval), as well as re-ranking via a cross-encoder to retrieve the most relevant data.
(2) Cleaning the data: The data used for RAG may come from several sources with different formats (e.g., pdf, markdown and more), which could lead to artifacts (e.g., logos, icons, special symbols, and code blocks) that could confuse the LLM. We can solve this by creating a data preprocessing or cleaning pipeline (either manually or by using LLM-as-a-judge) that properly standardizes, filters, and extracts data for RAG.
(3) Prompt engineering: Successfully applying RAG is not just a matter of retrieving the correct context—prompt engineering plays a massive role. Once we have the relevant data, we must craft a prompt that i) includes this context and ii) formats it in a way that elicits a grounded output from the LLM. First, we need an LLM with a sufficiently large context window. Then, we can adopt strategies like diversity and lost-in-the-middle selection to ensure the context is properly incorporated into the prompt.
(4) Evaluation: We must also implement repeatable and accurate evaluation pipelines for RAG that capture the performance of the whole system, as well as its individual components. We can evaluate the retrieval pipeline using typical search metrics (DCG and nDCG), while the generation component of RAG can be evaluated with an LLM-as-a-judge approach. To evaluate the full RAG pipeline, we can also leverage systems like RAGAS.
(5) Data collection: As soon as we deploy our RAG application, we should begin collecting data that can be used to improve the application. For example, we can finetune retrieval models over pairs of input queries with relevant textual chunks, finetune the LLM over high-quality outputs, or even run AB tests to quantitatively measure if changes to our RAG pipeline benefit performance.
What’s next? Beyond the ideas explored above, there are a variety of avenues that exist for improving RAG. Once we have implemented a robust evaluation suite, we can test a variety of improvements using both offline metrics and online AB tests. Our approach to RAG should mature (and improve!) over time as we test new ideas.
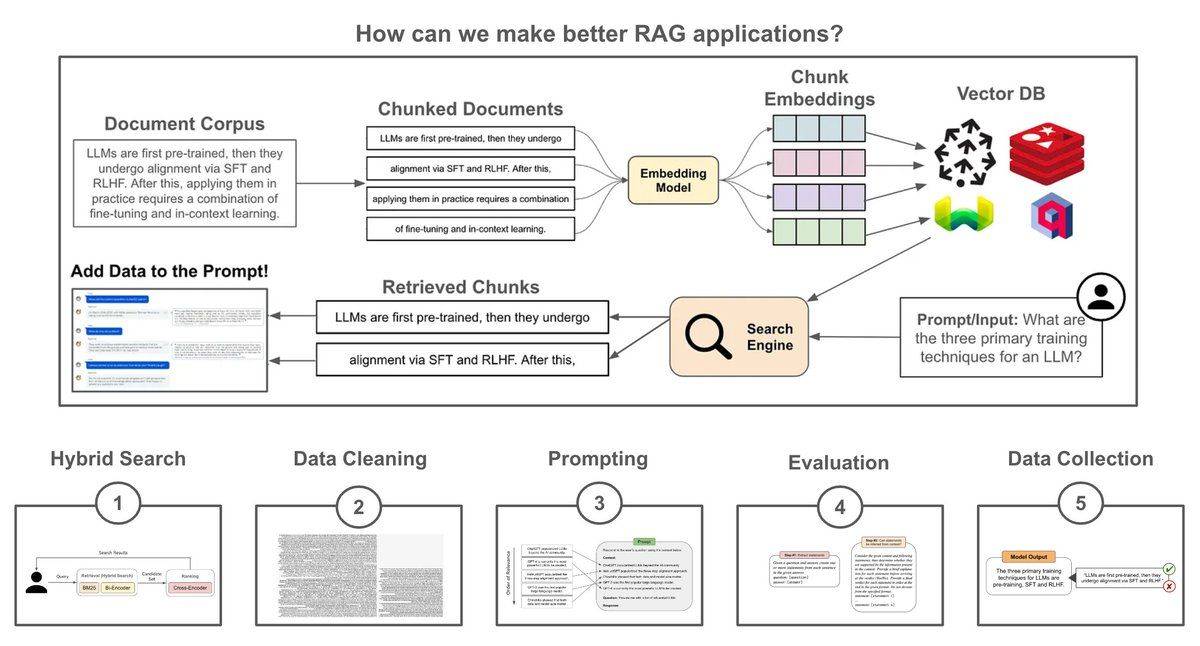