包阅导读总结
1.
关键词: 金融机构、监管数据管理、风险分析、数据智能平台、挑战与应对
2.
总结:金融机构面临监管和风险管理难题,从旧系统转向云时面临数据安全、架构复杂和迁移成本等挑战。Databricks 数据智能平台可解决这些问题,提供数据管理、治理和风险模型实施等能力,助力提升效率、灵活性和可扩展性。
3.
主要内容:
– 金融机构面临监管和风险管理挑战
– 现有系统无法满足需求,复杂风险流程是障碍
– 应对挑战的举措
– 关注数据管理和治理、风险模型实施和执行
– 转向云,但存在数据安全等三大挑战
– Databricks 数据智能平台的作用
– 解决监管风险和金融的数据管理与治理问题
– 统一数据格式、保证数据质量等
– 助力风险模型实施和执行
– 优化数据访问、管理模型生命周期等
– 平台优势
– 提升效率、灵活性和可扩展性
– 呼吁金融机构采用以满足监管风险管理需求
思维导图:
文章来源:databricks.com
作者:Databricks
发布时间:2024/6/28 12:10
语言:英文
总字数:1098字
预计阅读时间:5分钟
评分:84分
标签:行业,金融服务
以下为原文内容
本内容来源于用户推荐转载,旨在分享知识与观点,如有侵权请联系删除 联系邮箱 media@ilingban.com
Introduction
Financial institutions face a demanding environment with complex regulatory examinations and a pressing need for flexible and comprehensive risk management solutions. The burden of outdated infrastructure and isolated data for different types of risk makes existing systems unable to provide the necessary data framework for sustainable and efficient compliance with regulatory expectations across CECL, Stress Testing, Liquidity Risk, and Regulatory Data Reporting. Furthermore, complex risk processes have long been significant obstacles, hindering quick adaptation to changing regulations and efficient data processing and analysis.
In response to these challenges, financial institutions are increasingly shifting their focus from outdated legacy systems to the cloud. This shift is a crucial driver of innovation and operational efficiency, particularly for integrating risk and finance functions. Financial institutions can consider two important initiatives to address regulatory concerns when modernizing risk management.
- Data Management and Governance for Regulatory Risk and Finance: Focusing on enhancing control and oversight to meet compliance needs.
- Risk Model Implementation and Execution: Streamline processes for credit risk model implementation and execution.
Despite these areas of clear opportunities, there is still hesitation due to three main challenges:
- Data Security and Governance: Migrating sensitive financial data to the cloud raises critical questions about security, data access, and control, especially under the financial sector’s stringent regulatory standards.
- Architectural Complexity: Financial institutions are entangled in a web of on-premise legacy systems, complicating their cloud integration. The opaque logic of these systems heightens concerns during migration.
- Cost of Migrating to the Cloud: Transitioning to the cloud entails considerable upfront investment, including expenses for data migration, re-architecting applications, and staff training. This necessitates a well-thought-out strategy.
Many Financial institutions have successfully addressed these challenges using the Databricks Data Intelligence Platform. The platform integrates risk data, finance data, and risk analytics processes into a single platform, and Generative AI capabilities provide deep insights into each institution’s unique data and analytics ecosystem. This blog post outlines how the Databricks Data Intelligence Platform can serve as a modern solution for risk management, focusing on two foundational requirements: Data Management and Governance for Regulatory Risk and Risk Model Implementation and Execution.
Data Management and Governance for Regulatory Risk and Finance
Risk management solutions consume and forecast data across multiple levels of hierarchy and granularity, tailored to specific portfolios, credit risk, market risk, counterparty credit risk, ALM, liquidity risk, regulatory capital, and regulatory reporting. Given the various legacy technologies, such as SAS, Oracle, SQL Server, and Hadoop, challenges frequently arise during regulatory audits. These challenges are particularly evident in demonstrating the ability to trace data back to its sources, maintain accuracy, and uphold reliable data governance. The Databricks Data Intelligence Platform solves these issues with:
- Delta Lake UniForm: Delta Lake UniForm unifies diverse data formats and types, ensuring data reliability and integrity for risk analytics solutions and regulatory reporting.
- Delta Live Tables for Data Quality: For regulatory risk, where data accuracy and consistency are critical, Delta Live Tables ensure validation of all required data fields. The platform’s monitoring capabilities also provide comprehensive data quality metrics reporting.
- Unity Catalog for Consistent Data Compliance Management: Unity Catalog delivers robust data governance with secure access, clear lineage, and consistent data controls, forming the foundation for precise and integrated risk management.
- Workflow Orchestration for Reliable SLAs: Databricks Workflows enhance the orchestration and execution of data pipelines for analytics, ETL, reconciliation, and sharing, ensuring consistent SLA delivery and bolstering reliability during high regulatory demand.
The Data Intelligence Platform simplifies regulatory examinations by consolidating risk and finance data initiatives and enabling advanced analytics capabilities on a single platform without needing multiple vendors.
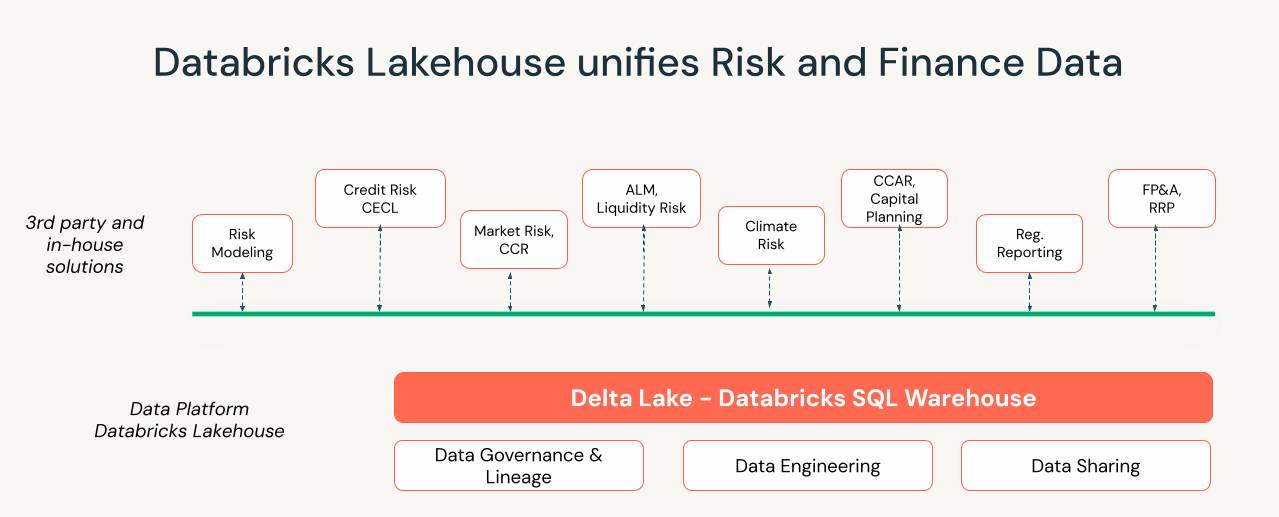
Additionally, the following features provide various enhancements for data management in regulatory risk management (highlighted in blue):
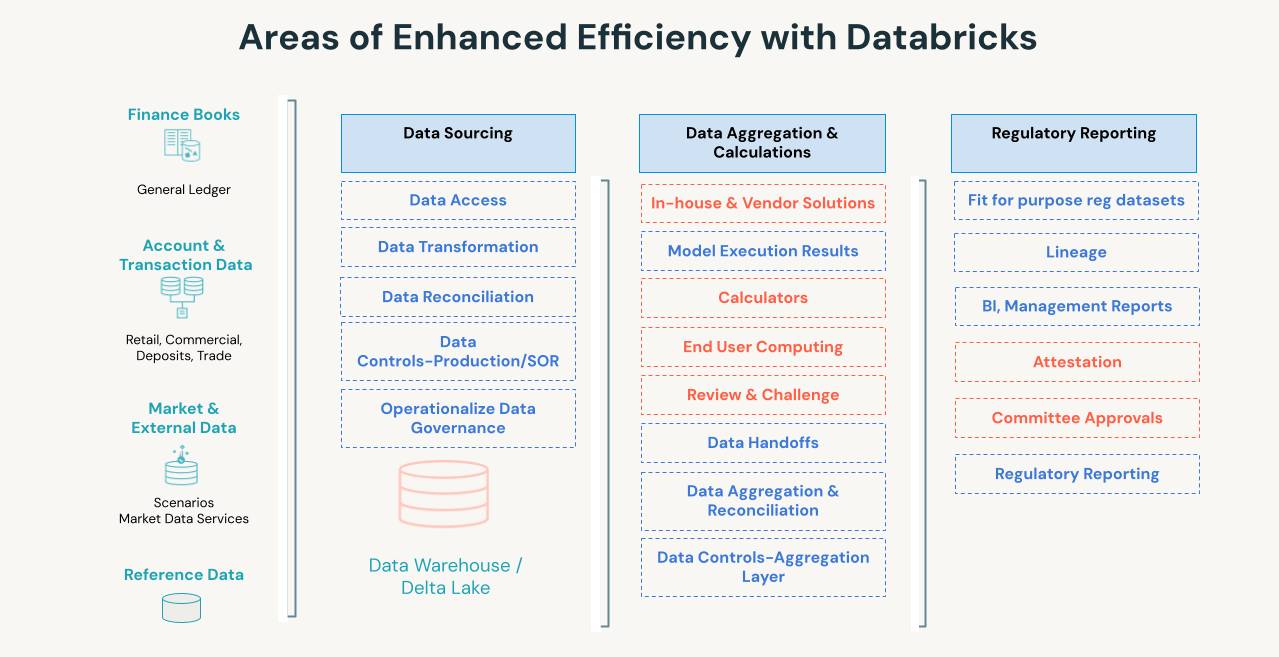
Risk Model Implementation and Execution
Every Financial institution has its unique framework for implementing and executing credit loss forecasting models, which are crucial for capital planning, stress tests, and CECL compliance. These frameworks often use models in different programming languages such as SAS, R, and Python. They are implemented across various platforms, including SAS Grid, open-source platforms, purpose-built solutions, or within ALM systems. Challenges with a lack of agility, resource-intensive processes, and complex risk process integration make it difficult to adapt to rapidly evolving macroeconomic and geopolitical changes, incorporate new data sources, and meet regulatory demands within model implementation and execution frameworks.
The Databricks Data Intelligence Platform offers comprehensive capabilities for model lifecycle management, implementation, and execution. This enhances risk management and capital planning, fostering a more dynamic and responsive risk management framework that equips financial institutions to face present and future regulatory demands efficiently. Below are a few capabilities that facilitate model implementation and execution.
- Lakehouse Federation: Streamlines data access with Lakehouse Federation, whether on-premises or in the cloud.
- MLflow for Model Lifecycle Management: Manages model versions and streamlines the model implementation, governance, and deployment process.
- Workflow Orchestration for Model Execution: Databricks Workflows orchestrates and automates the end-to-end execution, from start point reconciliation and scenario analysis to report review and challenge processes. This enables agile and scalable methods for executing various scenarios and portfolios.
- Unity Catalog for Lineage and Governance: Provides end-to-end lineage and complete controls, reinforcing adherence to regulatory requirements and ensuring fewer regulatory issues. Monitors data pipelines and model drift/back-testing to ensure the accuracy of results.
- Delta Sharing for Integration:Promotes data sharing and maintains critical integrations with systems like Asset and Liability Management, Financial Planning and Analysis, and Capital Planning, while supporting gradual cloud adoption and enhancing collaboration between risk and finance departments.
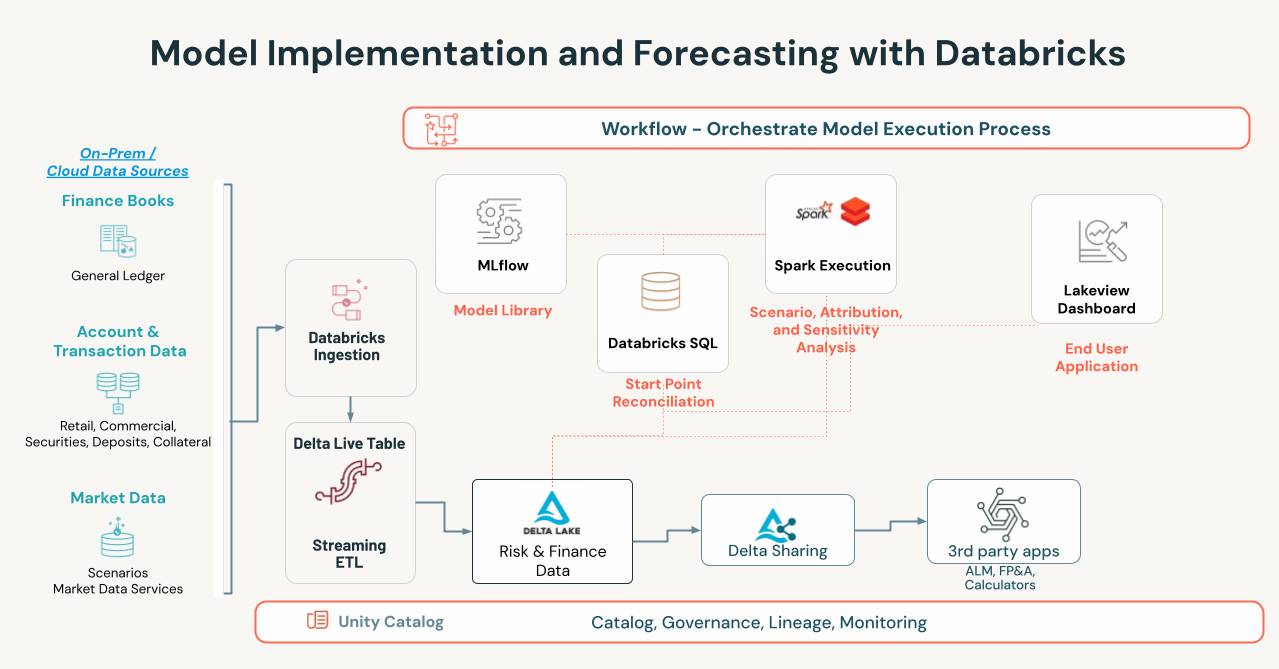
While the model implementation requirements vary for different organizations, the core capabilities we listed above should enable financial institutions to run their regulatory workloads on Databricks, simplify their architecture, address technical challenges, and align with regulatory compliance.
Unlock Efficiency, Agility, and Scalability
Enhance your regulatory reporting processes by leveraging the robust Databricks Data Intelligence Platform. Utilize Databricks’ features to build dependable data pipelines that efficiently intake, validate, and handle regulatory data by strict SLAs. Take advantage of integrated data lineage, governance, and security, fostering strong assurance in the accuracy and timeliness of your regulatory submissions. Effortlessly incorporate resilient model deployment and flexible forecasting into your operations on a unified platform.
Take the next step in modernizing your regulatory data management, risk analytics, and reporting processes. Ready to elevate your financial institution’s capabilities? Contact us today to unlock the full potential of a centralized data and analytics platform tailored to meet all your regulatory risk management needs.