包阅导读总结
1.
关键词:MTIA、Meta、co-design、AI 工作负载、模型架构
2.
总结:Meta 的软件工程师 Joel Colburn、技术主管 Junqiang Lan 和软件工程师 Jack Montgomery 在 AI Infra @ Scale 2024 中讨论了 MTIA 的第二代,涵盖其为 AI 工作负载构建的协同设计过程,包括软件生态和模型架构,展示了如何实现性能等以成功投入生产,并强调利用特殊硅片特征加速模型的协同设计示例。
3.
主要内容:
– 讨论 MTIA 的第二代,Meta 内部的训练和推理加速器
– 介绍参与讨论的人员
– 涉及 Meta 首款定制硅片
– 协同设计过程
– 针对 AI 工作负载
– 涵盖 PyTorch 软件生态系统
– 包含 Meta 关键应用的模型架构
– 成果展示
– 实现性能、效率和良好的开发者体验
– 成功将模型投入生产
– 列举利用特殊硅片特征加速模型的协同设计例子
思维导图:
文章地址:https://engineering.fb.com/2024/08/22/ml-applications/meta-mtia-hardware-co-design/
文章来源:engineering.fb.com
作者:Engineering at Meta
发布时间:2024/8/20 23:22
语言:英文
总字数:101字
预计阅读时间:1分钟
评分:84分
标签:AI 硬件,协同设计,Meta,MTIA,PyTorch
以下为原文内容
本内容来源于用户推荐转载,旨在分享知识与观点,如有侵权请联系删除 联系邮箱 media@ilingban.com
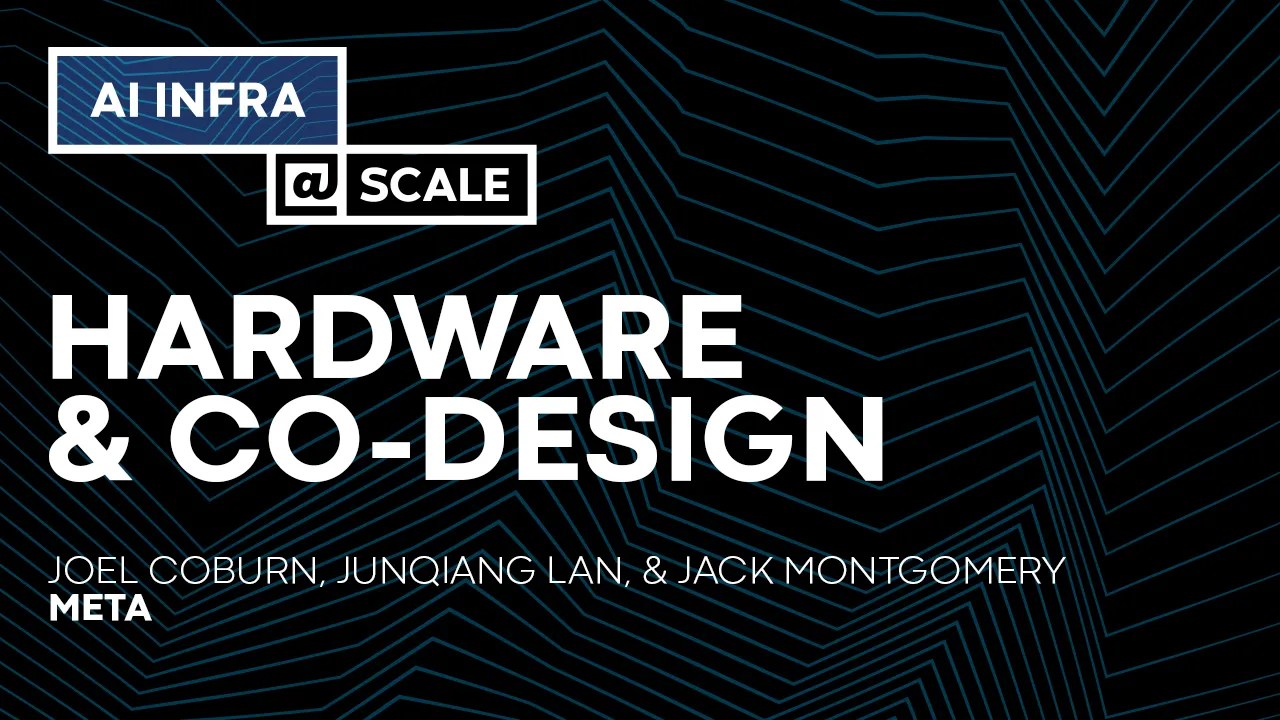
In this talk from AI Infra @ Scale 2024, Joel Colburn, a software engineer at Meta, technical lead Junqiang Lan, and software engineer Jack Montgomery discuss the second generation of MTIA, Meta’s in-house training and inference accelerator.
They cover the co-design process behind building the second generation of Meta’s first-ever custom silicon for AI workloads, including the PyTorch software ecosystem, and the model architectures for Meta’s key applications. They demonstrate how MTIA achieves the performance, efficiency, and developer experience to successfully launch models into production. They also highlight several co-design examples where special silicon features are utilized to accelerate Meta’s models.
VIDEO