包阅导读总结
1. 关键词:LLMs、Decoder-only Transformer、Architecture、Attention、Feed-forward
2. 总结:LLMs 发展迅速但底层架构变化不大,其中解码器仅转换器架构多年未变,是 AI 研究的重要基础,文章介绍了其五个组件。
3. 主要内容:
– LLMs 发展与架构:
– 新语言模型不断推出,但大多采用类似原始 GPT 的解码器仅转换器架构。
– 解码器仅转换器架构的重要性:
– 是 AI 研究中最重要的想法之一,值得深入理解。
– 架构的五个组件:
– 输入层:将文本提示转换为 token 向量,可添加位置嵌入。
– 因果自注意力:是核心,只计算序列中先前 token 的注意力分数,多头并行。
– 前馈变换:在每个块内对 token 表示进行变换。
– 分类头:从最终输出层接收 token 向量,输出与词汇表大小相同的向量用于训练或推理。
– 转换器块:包含因果自注意力和前馈变换,有层归一化模块和残差连接。
思维导图:
文章地址:https://stackoverflow.blog/2024/08/22/llms-evolve-quickly-their-underlying-architecture-not-so-much/
文章来源:stackoverflow.blog
作者:Cameron R. Wolfe,PhD
发布时间:2024/8/22 16:25
语言:英文
总字数:484字
预计阅读时间:2分钟
评分:84分
标签:大型语言模型 (LLMs),Transformer 架构,人工智能研究,仅解码器 Transformer,人工智能技术
以下为原文内容
本内容来源于用户推荐转载,旨在分享知识与观点,如有侵权请联系删除 联系邮箱 media@ilingban.com
New language models get released every day (Gemini-1.5, Gemma, Claude 3, potentially GPT-5 etc. etc.), but one component of LLMs has remained constant over the last few years—the decoder-only transformer architecture.
Why should we care? Research on LLMs moves fast. Shockingly, however, the architecture used by most modern LLMs is pretty similar to that of the original GPT model. We just make the model much larger, modify it slightly, and use a more extensive training (and alignment) process. For this reason, the decoder-only transformer architecture is one of the most fundamental/important ideas in AI research, so investing into understanding it deeply is a wise idea.
This architecture has five components:
(1) Input layer: Decoder-only transformers receive a textual prompt as input. We use a tokenizer—based upon an algorithm like Byte-Pair Encoding (BPE)—to break this text into discrete tokens (i.e., words or sub-words). Then, we map each of these tokens to a corresponding vector stored in an embedding layer. This process forms a sequence of token vectors that are passed to the model as input. Optionally, we can augment these token vectors with additive positional embeddings.
(2) Causal self-attention is the core of the decoder-only transformer and allows the model to learn from relationships between tokens in the input. The vanilla self-attention operation transforms each token’s representation by taking a weighted combination of other token representations, where weights are given by pairwise attention/importance scores between tokens. Causal self-attention follows a similar strategy but only computes attention scores for preceding tokens in the sequence. Attention is performed in parallel across several heads (i.e., multi-head attention), each of which can focus upon different parts of the input sequence.
(3) Feed-forward transformations are performed within each block of the decoder-only transformer, allowing us to individually transform each token’s representation. This feed-forward component is a small neural network that is applied in a pointwise manner to each token vector. Given a token vector as input, we pass this vector through a linear projection that increases its size by ~4X, apply a non-linear activation function (e.g., SwiGLU or GeLU), then perform another linear projection that restores the original size of the token vector.
(4) Classification head: The decoder-only transformer has one final classification head that takes token vectors from the transformer’s final output layer as input and outputs a vector with the same size as the vocabulary of the model’s tokenizer. This vector can be used to either train the LLM via next token prediction or generate text at inference time via sampling strategies like nucleus sampling and beam search.
(5) Transformer blocks form the body of the decoder-only transformer architecture. The exact layout of the decoder-only transformer block may change depending upon the implementation, but two primary sub-layers are always present:
1. Causal self-attention
2. Feed-forward transformation
Additionally, these sub-layers are surrounded by a layer normalization module—either before or after the sub-layer (or both!)—as well as a residual connection.
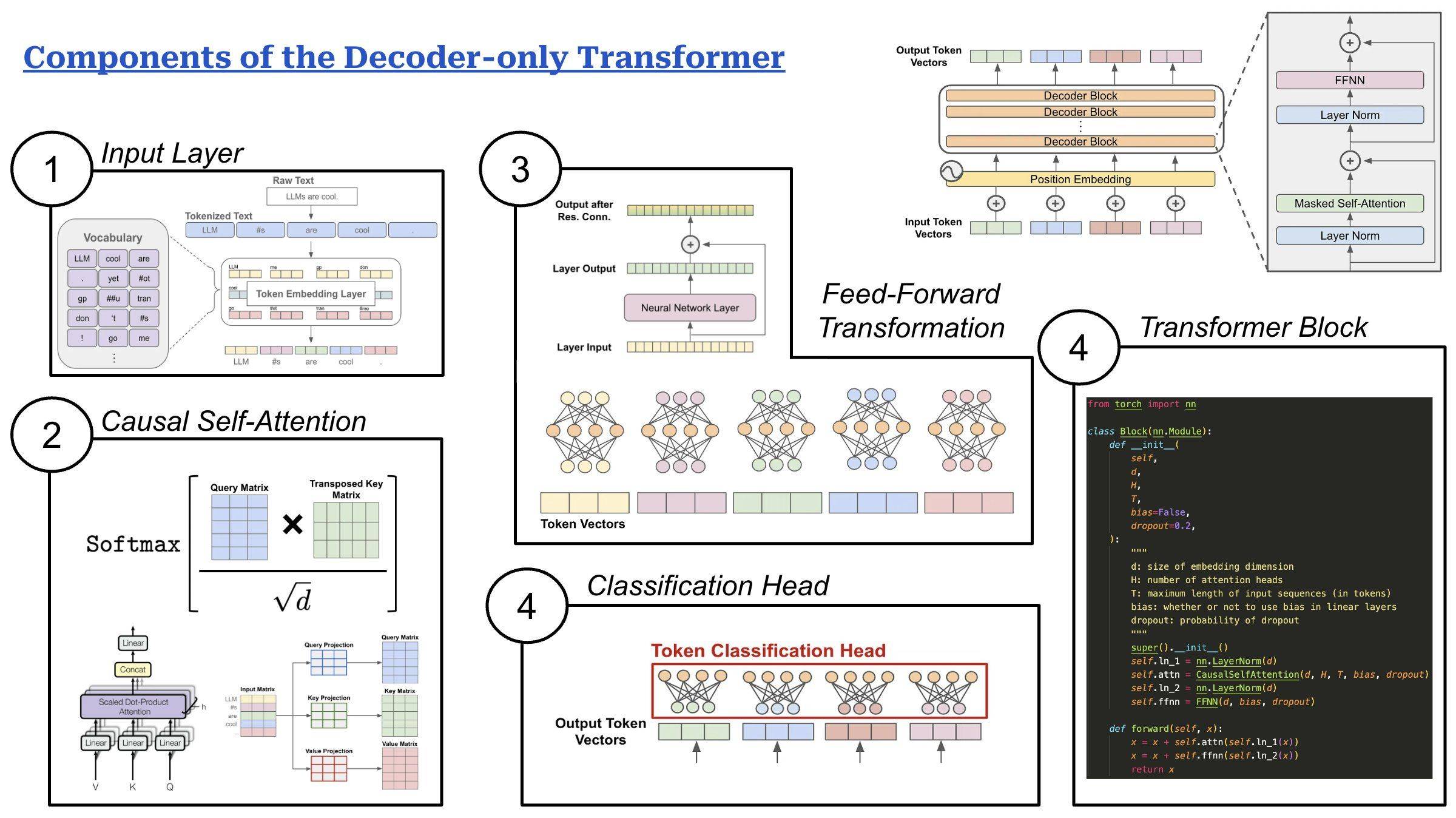