包阅导读总结
1. 关键词:Euro 2024、Python、数据分析、足球、JetBrains Academy
2. 总结:本文以 Euro 2024 足球赛事为例,介绍如何用 Python 进行数据分析,包括准备数据、分析球员进球身体部位、进球和黄牌与球衣号码关系、进球与星座的关联等,还提及相关学习课程和资源。
3. 主要内容:
– 准备数据:
– 说明数据来源为 Euro 2024 数据集。
– 介绍使用 PyCharm 及相关库,如 matplotlib 和 numpy 。
– 进球身体部位分析:
– 统计进球按身体部位的数量。
– 用饼图展示分析结果。
– 进球和黄牌与球衣号码关系:
– 准备获取对应事件球衣号码的函数。
– 绘制进球和黄牌的球衣号码直方图。
– 进球与星座的关联:
– 计算球员星座,分析不同星座进球情况。
– 课程与资源:
– 介绍 Football Analysis 项目。
– 推荐 Data Analyst 课程和 Introduction to Data Science 课程。
思维导图:
文章地址:https://blog.jetbrains.com/education/2024/08/09/euro-2024-scoring-goals-with-python/
文章来源:blog.jetbrains.com
作者:Regina Muradova
发布时间:2024/8/9 9:14
语言:英文
总字数:1364字
预计阅读时间:6分钟
评分:87分
标签:Python,数据分析,2024 年欧洲杯,足球统计,PyCharm
以下为原文内容
本内容来源于用户推荐转载,旨在分享知识与观点,如有侵权请联系删除 联系邮箱 media@ilingban.com
Euro 2024: Scoring Goals With Python
Now that the 2024 UEFA European Football Championship is over, and we’ve all experienced the thrilling moments of cheering for our favorite teams, let’s have some fun with Python and dive into the tournament’s data. Why not combine our love for football with some cool data analysis?
In this blog post, we’ll demonstrate how Python works by exploring data from Euro 2024. Not only will we gain new insights about Europe’s premier sporting event, but we’ll also show you how to use Python for basic data analysis in real-world scenarios. And you may just find that data analysis can be as fun as the game itself.
Preparing the data
First, we need to set up our environment and load the data. The data for this analysis is sourced from the Euro 2024 dataset available from Kaggle. We took the 2024.csv file from the matches | euro
tab.
For this analysis, we’ll be using PyCharm. Either PyСharm Community Edition (completely free) or PyСharm Professional (available with a 30-day free trial) will be fine. Once you’ve downloaded the IDE, open it up, start a new project, and put the CSV file into the project folder. Make sure the matplotlib
and numpy
libraries are installed in your IDE.
We’ll run the code in Jupyter Notebook (available only in PyCharm Professional), but you can also run the code directly in PyCharm without the notebook—it’s entirely up to you.
▶︎ Basic setup and general functions necessary for the following analysis (click here to see the code👇🏻)
import csvimport matplotlib.pyplot as pltimport numpy as npimport matplotlibmatplotlib.use('TkAgg')CSV_PATH = '2024.csv'def load_csv(csv_path): """Load a CSV file and return two lists: events and players""" events = [] players = [] with open(csv_path, encoding='latin-1') as csv_f: fixed_data = csv_f.read().replace(' nan', ' None') # Preprocess data from csv-file reader = csv.reader(fixed_data.splitlines()) headers = next(reader) # Skip the header for row in reader: events += eval(row[-1]) players += eval(row[-4]) # Add teams from "home" games players += eval(row[-5]) # Add teams from "away" games # So far, the "players" list contains multiple copies of the same players from different games; # Fix it by putting the players into a dictionary by ID, thus overwriting duplicates players_dict = {i['id_player']: i for i in players} players = players_dict.values() # We're only interested in events related to a person (e.g. "goal", "card"). Remove the rest events = [i for i in events if i['primary_id_person'] is not None] return events, playersdef find_player_by_id(players, player_id): """Return the player corresponding to the specified player ID""" return [i for i in players if i['id_player'] == player_id][0]def filter_events_by_type(all_events, event_type): """Return a list of events with only a given type (e.g. 'GOAL')""" return [i for i in all_events if i['type'] == event_type]
Now let’s run the function def load_csv(csv_path)
to load data from the file:
events, players = load_csv(CSV_PATH)
We have loaded the data about events and players, and it’s now ready for analysis.
Most valuable body part
Ever wondered whether players score more with their head, right foot, or left foot? To kick off our analysis, we broke down goals by body parts. We collected the data and visualized it in a pie chart to see which body parts are the true game-changers on the field.
Counting goals by body part
def count_goals_by_body_part(goal_events): """Take goal events and count the number of goals per body part; return as a dict""" result = {} for event in goal_events: body_part = event.get('body_part') if body_part: result[body_part] = result.get(body_part, 0) + 1 return result
Displaying the pie chart
def show_body_part_pie_chart(body_parts_count): """Plot and show a pie chart of goals by body part""" colors = ['#AE1DF4', '#01E08F', '#7463FF', '#111111'] plt.figure(figsize=(6, 6), dpi=130) plt.pie( body_parts_count.values(), labels=body_parts_count.keys(), autopct='%1.1f%%', textprops={'color': '#333333'}, colors=colors[:len(body_parts_count)] # Adjust colors list length ) plt.title('Goals per body part') plt.show()
Running the functions
goal_events = filter_events_by_type(events, 'GOAL')body_parts_count = count_goals_by_body_part(goal_events)show_body_part_pie_chart(body_parts_count)
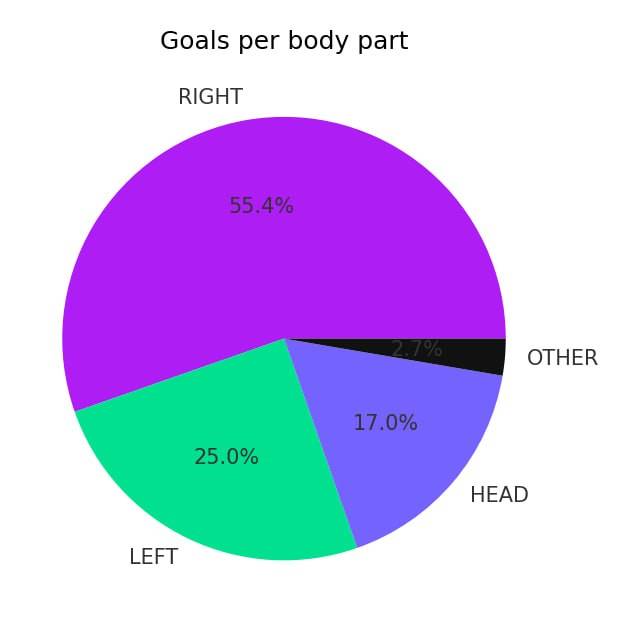
Our analysis showed some expected results, with right-footed goals being the most common. However, it also revealed that left-footed goals were more frequent than headers, showing that in football, each of these body parts can make a difference when it comes to scoring goals.
Watch the demo to see how this code runs in the IDE. 👇🏻
Goals and yellow cards by jersey numbers
Next, we looked at goals scored by players based on their jersey numbers. Do players with certain numbers tend to score more goals? Let’s find out.
Preparing the functions
def get_jerseys_numbers_by_event(events, players, event_type): """Return a simple list of jersey numbers corresponding to events of specified event_type""" selected_events = filter_events_by_type(events, event_type) # Helper function to find a player by ID and return his number def jersey_num_by_id(player_id): return find_player_by_id(players, player_id)['jersey_namber'] return [jersey_num_by_id(i['primary_id_person']) for i in selected_events] def plot_jerseys_numbers_histogram(jerseys_list, data_label, data_color): '''Plot the jersey histogram based on "jerseys_list" data''' MAX_JERSEY_NUM = 26 _, bins, _ = plt.hist( jerseys_list, bins=np.arange(MAX_JERSEY_NUM + 2) - 0.5, label=data_label, edgecolor='black', color=data_color, alpha=0.5 ) plt.xticks(0.5 * (bins[1:-1] + bins[2:])) # Make sure all numbers are labeld on x-axis plt.legend()
Running the functions and displaying the histograms
goals_jerseys = get_jerseys_numbers_by_event(events, players, 'GOAL')cards_jerseys = get_jerseys_numbers_by_event(events, players, 'YELLOW_CARD') plt.subplot(2, 1, 1) # Top plotplt.title('Events by jersey number')plot_jerseys_numbers_histogram(goals_jerseys, 'Goals', 'lightgreen') plt.subplot(2, 1, 2) # Bottom plotplt.xlabel('Jersey number')plot_jerseys_numbers_histogram(cards_jerseys, 'Yellow cards', 'yellow') plt.show()
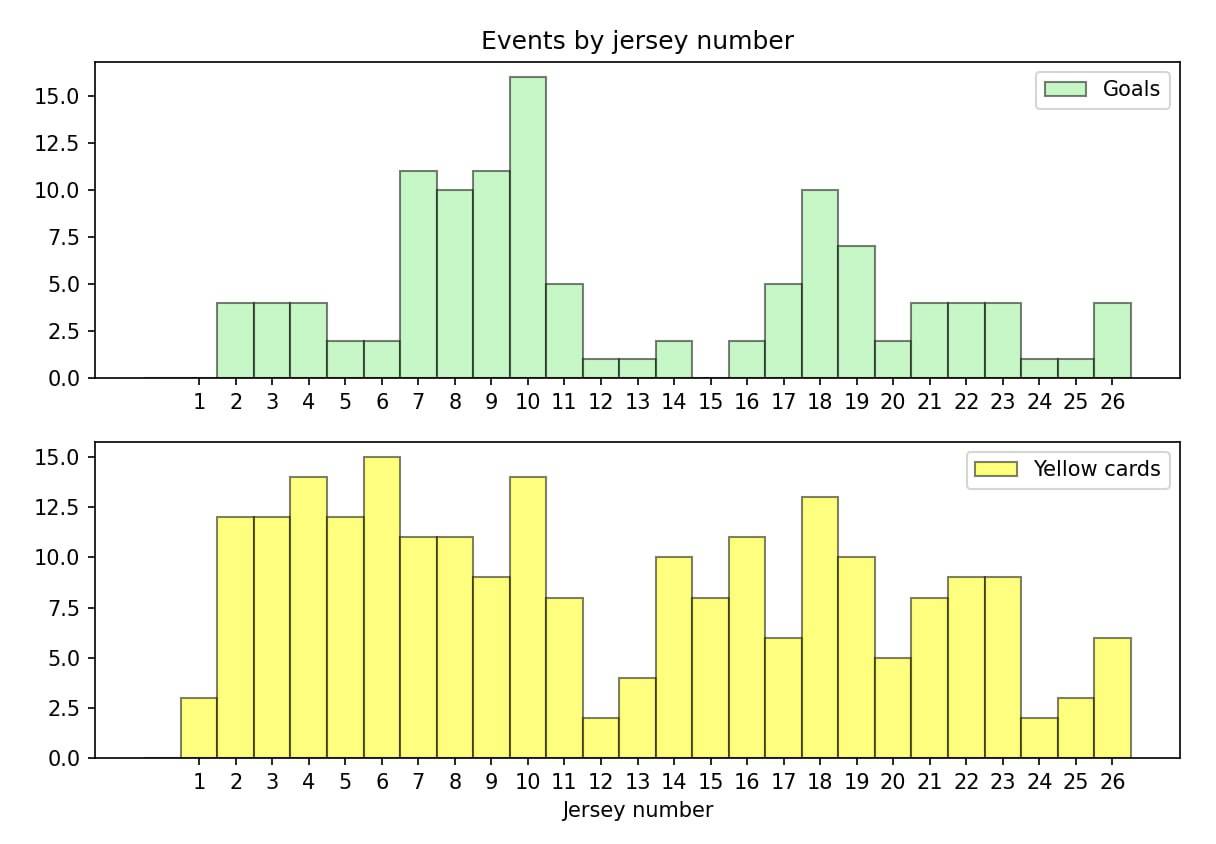
Watch the demo to see how this code runs in the IDE. 👇🏻
Our analysis revealed that players wearing the number 10 jersey scored the most goals. Legends like Pelé, Maradona, and Messi all wore this iconic number, demonstrating that the greatest forwards truly prefer number 10.
We also looked at which jersey numbers get the most yellow cards. Defenders wearing numbers like 4 and 6 rack them up the most. Are defensive responsibilities more likely to land you on the wrong side of the ref’s whistle? Or do players with a penchant for flouting the rules simply gravitate toward defense? It’s hard to say from these numbers alone.
Numbers like 1, 12, and 24 show up less frequently for both goals and yellow cards. This could be due to these numbers often being worn by goalkeepers.
Using Python, we visualized these trends with histograms. Some numbers, like 10, stand out with scoring goals, while others, like 4 and 6, handle the tougher tasks on the field.
Goals by zodiac signs
And finally, we thought it would be fun to see if Python could tell us anything about football players based on their zodiac signs. Could the stars really predict on-field actions? In this playful experiment, we mixed astrology with football to find out.
We started by calculating the zodiac sign for each player using their birth date. Then, we looked at how often players with different signs scored goals or received yellow cards. After adjusting for the overall distribution of zodiac signs among players in each game, we visualized the data with some cool histograms. We won’t dive into the code details here—instead, we invite you to simply enjoy the chart.
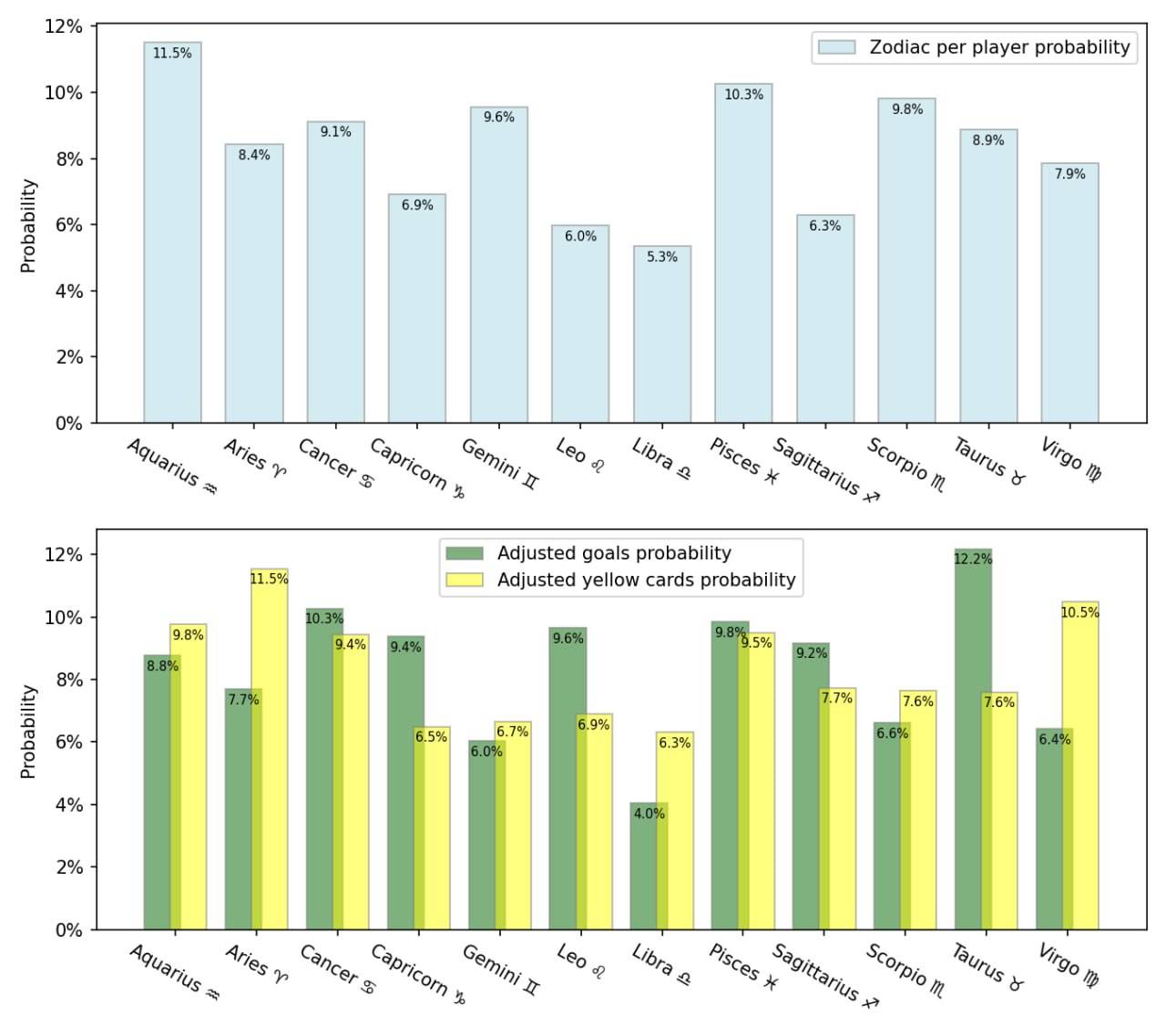
Take a look at your sign on the chart – do the stats align with its profile? Taurus players, who are known for being determined and steady, seem to score the most goals. On the other hand, Aries, with their fiery and bold nature, are leading in yellow cards.
As you can see, there’s no limit to the types of interesting insights you can uncover with Python and data science. If football isn’t your thing, what other data would you like to analyze with Python?
If you also want to try Python, you can dive into our newFootball Analysisproject, where you’ll get hands-on experience analyzing football data. Or if you are eager to learn data analysis and kickstart your career in this field, explore our Data Analyst course on Hyperskill. You’ll learn how to handle, visualize, and interpret data effectively. For a deeper understanding of data analysis, consider adding Introduction to Data Science to your portfolio.
Happy learning!
Your JetBrains Academy team
Note: The data used in this analysis is for educational purposes only and is not intended to bring JetBrains commercial advantage or monetary compensation. For further use of any data mentioned in this post, please always ensure you comply with the relevant license terms.