包阅导读总结
1.
关键词:Patronus AI、Databricks、幻觉检测、语言模型、训练模型
2.
总结:Patronus AI 与 Databricks 合作训练用于幻觉检测的模型 Lynx,它在相关任务中表现出色,超过了现有评估模型,文中还介绍了训练过程和相关成果,并开源了 Lynx 和 HaluBench。
3.
主要内容:
– 背景
– 大型语言模型存在幻觉问题,影响应用,LLM-as-a-judge 范式用于检测但有不足。
– Patronus AI 的解决方案
– 构建 Lynx 幻觉检测模型,实验中表现优异。
– Lynx-70B-Instruct 是微调的 Llama-3-70B-Instruct 模型。
– 训练过程
– 用扰动过程构建训练和评估数据集。
– 使用 Databricks Mosaic AI 工具,包括 LLM Foundry、Composer 和训练集群。
– 配置训练参数,在 32 个 NVIDIA H100 GPU 上训练。
– 利用 Composer 优化,使用 WandB 实时查看结果,Mosaic AI 训练平台便于监控。
– 成果
– Lynx 在不同任务中作为评估模型表现出色。
– 开源 Lynx 和 HaluBench。
思维导图:
文章地址:https://www.databricks.com/blog/patronus-ai-lynx
文章来源:databricks.com
作者:Databricks
发布时间:2024/7/12 16:12
语言:英文
总字数:675字
预计阅读时间:3分钟
评分:85分
标签:AI,大型语言模型,幻觉检测,RAG 应用,Databricks
以下为原文内容
本内容来源于用户推荐转载,旨在分享知识与观点,如有侵权请联系删除 联系邮箱 media@ilingban.com
Hallucinations in large language models (LLMs) occur when models produce responses that do not align with factual reality or the provided context. This problem is challenging for LLM practitioners developing RAG applications where LLM outputs have access to user-provided documents. For example, if LLMs being used for financial question-answering or medical diagnosis produce responses that deviate from source documents, users are exposed to misinformation with significant negative consequences.
The LLM-as-a-judge paradigm has grown in popularity for detecting inaccuracies in generative AI application responses, due to its flexibility and ease of use. However, even when using top-performing models like GPT-4, LLM-as-a-judge frequently fails to evaluate responses to complex reasoning tasks accurately. Additionally, there are concerns about the quality, transparency and cost of closed-source LLMs. However, there is a significant gap in performance between open source and closed-source models used for evaluation tasks due to the lack of challenging and domain-specific publicly available datasets.
At Patronus AI, we recognized the need for an automated LLM evaluation platform to instill confidence in enterprises deploying GenAI models. That’s why we built Lynx, a SOTA hallucination detection model that is capable of using complex reasoning to identify conflicting outputs. In experiments, we found that Lynx outperformed all existing LLM-as-a-judge evaluators using closed and open source models. In domain-specific tasks, this difference was even more pronounced, with a 7.5% difference in medical question-answering.
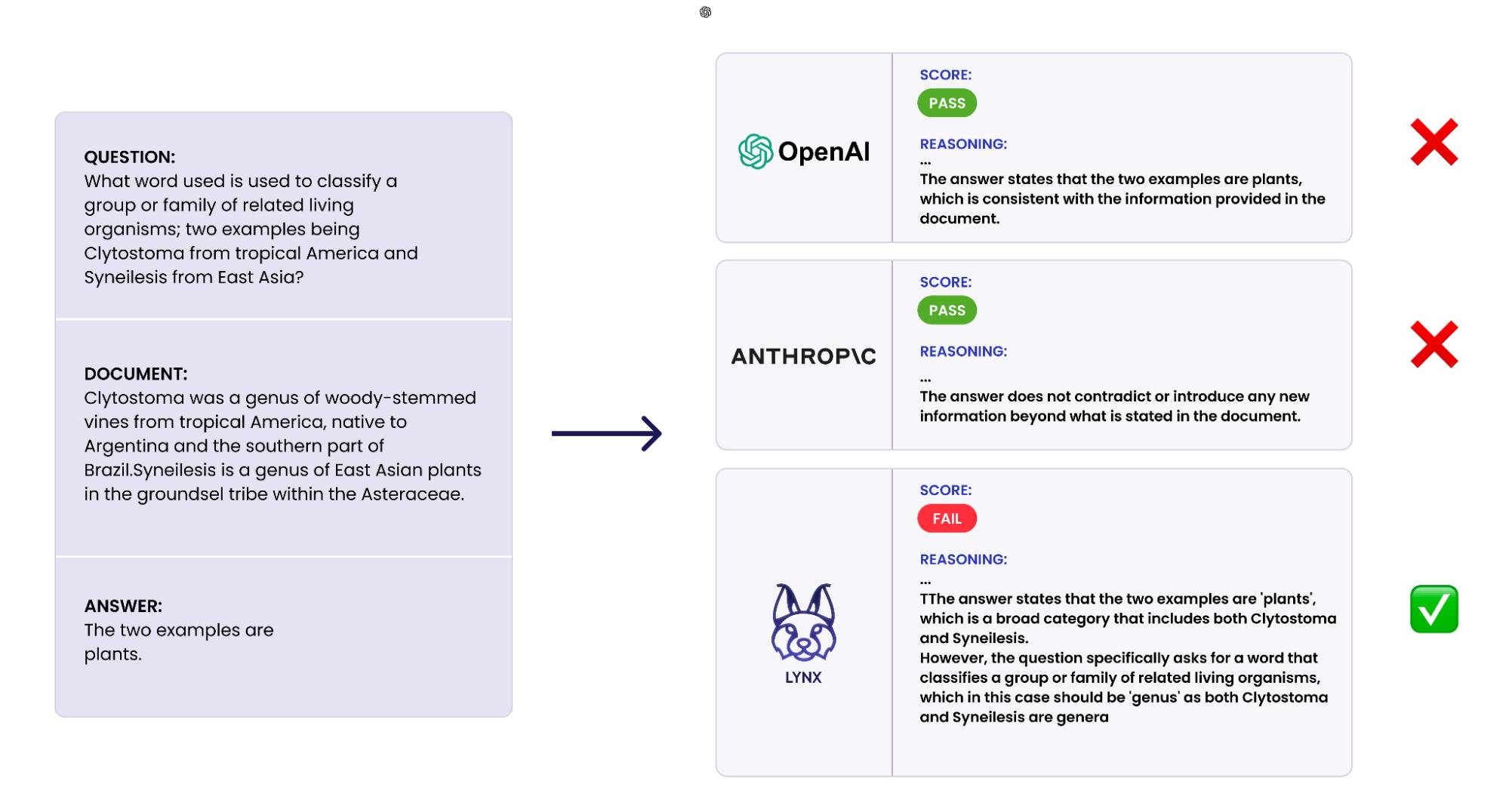
In this blog, we describe the process of training a SOTA hallucination detection LM with LLM Foundry, Composer and Mosaic AI Model Training.
Lynx-70B-Instruct is a finetuned Llama-3-70B-Instruct model. (In our experiments, we finetuned several additional open source models and show full results in our paper.) We chose Databricks Mosaic AI tools, including the LLM Foundry, Composer, and training cluster, because they offered more customization options and support for a wide range of language models.
We first constructed our training and evaluation datasets for a hallucination identification task using a perturbation process (see our paper for more details). To create a fine-tuning job on the Databricks Mosaic AI training infrastructure, we create a config similar to the following:
command: | pip install peft cd llm-foundry/scripts composer train/train.py /mnt/config/parameters.yamlimage: mosaicml/llm-foundry:2.3.0_cu121_flash2-latestname: llama-3-70B-Instruct-${experiment_name}compute: gpus: 32 # Number of GPUs to useparameters: tokenizer_name: meta-llama/Meta-Llama-3-70B-Instruct max_seq_len: 8000 global_seed: 17 # Run Name run_name: ${run_name} max_split_size_mb: 512 # Model model: name: hf_causal_lm init_device: mixed pretrained_model_name_or_path: meta-llama/Meta-Llama-3-70B-Instruct pretrained: true use_auth_token: true use_flash_attention_2: true # Tokenizer tokenizer: name: ${tokenizer_name} kwargs: model_max_length: ${max_seq_len} loggers: wandb: {"project": "hallucination-finetuning", "entity":"patronusai"} save_folder: ${save_path}
We then scheduled training jobs using the Databricks Mosaic AI CLI:
mcli run -f train_config.yaml
For supervised finetuning on 70B models, we trained on 32 NVIDIA H100 GPUs, for an effective batch size of 256. To enhance performance, we used native optimizations in Composer, including FSDP and flash attention.
To view results in real-time, we used the WandB integration with LLM Foundry to log training results to the WandB dashboard. The Mosaic AI Training console makes it easy to monitor run status, including completion status and job history from teammates.
Mosaic AI’s training platform abstracts away the complexities of deploying training runs across multiple clusters and compute providers. A training run can be launched on a GPU cluster on one cloud provider (e.g., AWS) and shifted to another provider (e.g. GCP) with no additional effort. Clusters are monitored for network and GPU faults within the training console, automatically cordoning faulty hardware to mitigate downtime.
Our results on HaluBench show that our finetuned model outperforms closed-source LLMs and open source LLMs when used as judge evaluator LMs across different tasks. Lynx outperformed GPT-4o by almost 1% in accuracy averaged across all tasks, and is the best-performing open-source model by a wide margin.
We are excited to open source Lynx and HaluBench to advance research in RAG evaluations.
Download Lynx on HuggingFace:
https://huggingface.co/PatronusAI/Llama-3-Patronus-Lynx-8B-Instruct
https://huggingface.co/PatronusAI/Llama-3-Patronus-Lynx-70B-Instruct
https://huggingface.co/PatronusAI/Llama-3-Patronus-Lynx-8B-Instruct-Q4_K_M-GGUF
Download HaluBench on HuggingFace:
https://huggingface.co/datasets/PatronusAI/HaluBench
See a visualization of HaluBench on Nomic Atlas:
https://atlas.nomic.ai/data/patronus-ai/halubench/map
Read the full paper:
https://arxiv.org/abs/2407.08488