包阅导读总结
1. 关键词:
– LangSmith
– Dynamic few-shot examples
– Model performance
– Dataset curation
– LLM applications
2. 总结:
LangSmith 推出动态少样本示例选择器,动态选择示例能改进应用性能。少样本提示常用于提升模型性能,但复杂应用面临诸多挑战,动态少样本提示可克服部分问题,它在 LangSmith 中集成,操作更简便,能优化数据集管理和 LLM 应用性能,目前处于封闭测试阶段。
3. 主要内容:
– 动态少样本示例与 LangSmith 数据集
– 推出动态少样本示例选择器
– 少样本提示提升应用性能,动态选择能进一步改进
– 优化模型性能的挑战
– 复杂应用需大量示例,存在窗口限制、成本和延迟问题
– 微调有效果但存在执行复杂、难更新等缺点
– LangSmith 中的动态少样本示例
– 基于用户输入动态选择 3 – 5 个示例,效果优于静态示例
– 集成到 LangSmith 中,一键索引数据集,获取相似示例
– 相比微调更易操作、易更新、所需基础设施少
– 其他信息
– 处于封闭测试,可申请等待名单
– 可参考详细技术文档和视频
– 可查看其他相关资源
思维导图:
文章地址:https://blog.langchain.dev/dynamic-few-shot-examples-langsmith-datasets/
文章来源:blog.langchain.dev
作者:LangChain
发布时间:2024/8/11 23:50
语言:英文
总字数:595字
预计阅读时间:3分钟
评分:91分
标签:动态少样本提示,LangSmith,数据集管理,LLM 应用程序性能,AI 优化
以下为原文内容
本内容来源于用户推荐转载,旨在分享知识与观点,如有侵权请联系删除 联系邮箱 media@ilingban.com
Today, we are launching dynamic few-shot example selectors as part of LangSmith. Few shot prompting is a common technique used to improve application performance. Dynamically selecting the examples for a few-shot prompt can yield further improvements. To do this, you need the ability to curate a dataset and to have the infrastructure to index and search over said dataset.
LangSmith already easily enables dataset curation. Now, with this new feature, you can index examples in your datasets with the click of a button. This lets you dynamically select the most relevant few-shot examples based on user input, allowing you to refine and improve LLM apps more efficiently.
The challenges of optimizing model performance
Few-shot prompting involves including example model inputs and desired outputs in the model prompt, which can greatly boost model performance on a wide range of tasks. Typically, developers use 3-5 few shot examples in a prompt to avoid overloading the context window with trivial information.
As your application grows in complexity, however, you may need hundreds or even thousands of examples to cover diverse end user needs. Including a large dataset of examples in every request may be too large to add to the context window. Even if it fits, it will increase token cost and latency.
When working with this many examples, finetuning is often the next option. But while finetuning can be powerful and yield good results if done properly, it also has some downsides. Finetuning is (a) relatively complex to execute, (b) hard to update with new examples, (c) requires specialized infrastructure (GPUs) and expertise (MLEs), and (d) lacks personalization (i.e. can’t easily tailor examples to users).
As a result, finetuning may be less suitable for personalized applications and rapid iterations. This is where dynamic few-shot prompting can come in handy.
Dynamic few-shot examples in LangSmith
Dynamic few-shot prompting can help you rapidly iterate and improve LLM application performance. With this technique, you still use a small set of 3-5 examples but dynamically select which examples to use based on user input. These examples cover the full range of options, which can outperform static datasets of 3-5 examples. The difference between “dynamic” and “static” few-shot prompting is that with “dynamic” prompting, you select examples to include in the prompt based on user input, while with “static” prompting, the same examples are used regardless of user input.
We’ve integrated dynamic few-shot prompting into LangSmith to streamline dataset management and enhance your LLM application performance. With just one click, you can index your dataset and call an endpoint with a new input to get back a list of examples most similar to the new input.
Compared to fine-tuning, dynamic few-shot prompting is (a) much easier to do technically, (b) easier to keep up-to-date, and (c) requires minimal specialized infrastructure. You can retrieve relevant examples based on user inputs, making it easy to iterate quickly on applications that you can also adapt and personalize.
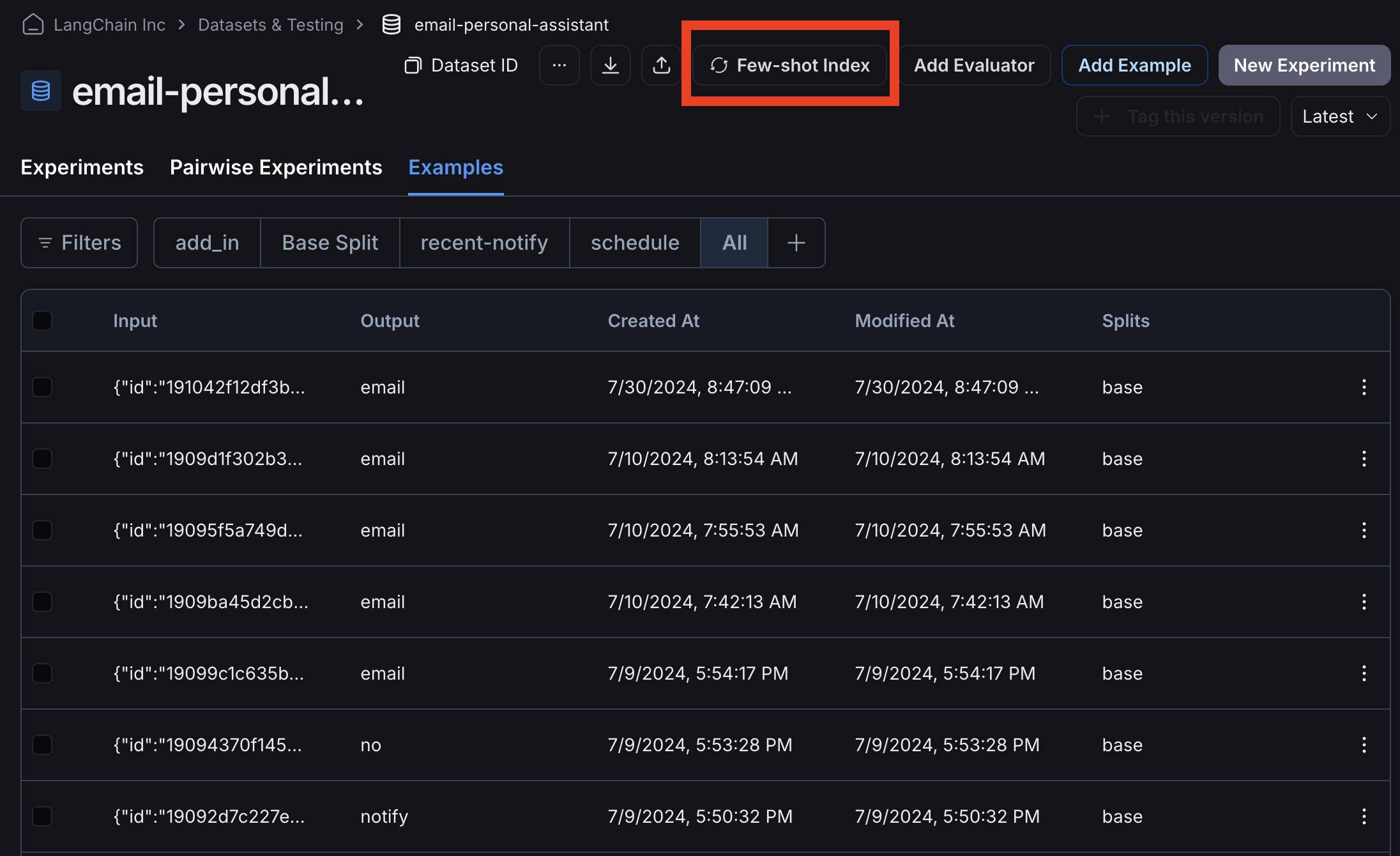
With dynamic few-shot prompting, you can streamline dataset management and refine your LLM app performance in LangSmith. Learn more on how to use dynamic few-shot prompting in our detailed technical documentation. You can also watch our video walkthrough below.
Dynamic few-shot prompting in LangSmith is currently in closed beta. You can sign up for the waitlist here. We are targeting a public launch later this month.
To start building a data flywheel to improve your LLM application, check out our other resources on managing data and testing in LangSmith, including:
Updates from the LangChain team and community