包阅导读总结
1. 关键词:`Dockerfile`、`GenAI`、`NPM 项目`、`生成`、`最佳实践`
2. 总结:本文探讨了在 Docker Labs GenAI 系列中利用 GenAI 生成 Dockerfile 的相关内容,介绍了为助手配备工具和遵循最佳实践来改进生成效果,包括分析项目、创建 Dockerfile 的流程,以及针对 NPM 项目的具体步骤,最后总结了关键因素并期待持续评估和改进。
3. 主要内容:
– 介绍 Docker Labs GenAI 系列将探索 AI 开发者工具领域
– 指出开发者对 AI 工具在软件全生命周期中辅助特定任务和接口的潜力
– 阐述让助手生成 Dockerfile 的方法,包括给予高级指令和提供函数定义
– 举例说明生成的 Dockerfile 存在的问题及针对 NPM 项目的最佳实践
– 展示改进后的输出及其中的改进因素
– 提及交流过程中的意外情况和未预设的应对
– 总结生成 Dockerfile 时工具和最佳实践的作用,并表示将继续评估对新类型项目的适应性
思维导图:
文章地址:https://www.docker.com/blog/how-to-create-dockerfiles-with-genai/
文章来源:docker.com
作者:Docker Labs
发布时间:2024/7/29 7:00
语言:英文
总字数:1643字
预计阅读时间:7分钟
评分:80分
标签:Docker,生成式AI,Dockerfile,AI 开发工具,DevOps
以下为原文内容
本内容来源于用户推荐转载,旨在分享知识与观点,如有侵权请联系删除 联系邮箱 media@ilingban.com
This ongoing Docker Labs GenAI series will explore the exciting space of AI developer tools. At Docker, we believe there is a vast scope to explore, openly and without the hype. We will share our explorations and collaborate with the developer community in real time. Although developers have adopted autocomplete tooling like GitHub Copilot and use chat, there is significant potential for AI tools to assist with more specific tasks and interfaces throughout the entire software lifecycle. Therefore, our exploration will be broad. We will be releasing things as open source so you can play, explore, and hack with us, too.
As we learn how to work more effectively with generative AI, one of the tasks we return to is Dockerfile generation. Because we can equip our AI assistants with tools, does this allow us to reframe the problem of content generation as an interaction between LLMs and tools?
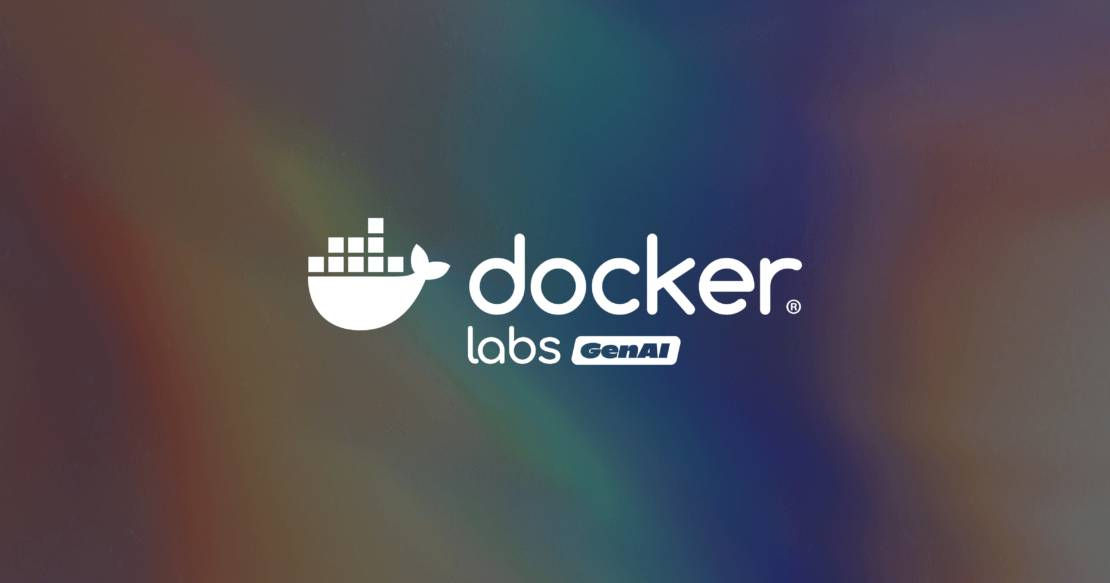
If you ask ChatGPT to write a Dockerfile for your project, it will initiate a conversation with you and ask you to extract some details from your project (Figure 1).
However, we can also equip our assistant with a tool to extract this information from the project directly. Instead of asking the user, an assistant can ask for a tool instead.
Using tools
Here’s an alternative framing. Let’s prompt the assistant with two high-level instructions whenever it is asked to create a Dockerfile.
* First, analyze the project to determine how it should be built. * Once the analysis is complete, create a Dockerfile to build that project.
In addition to these new prompts, we will also supply the LLM with two function definitions.
[{"name": "analyze_project", "description": "Analyze a project to determine how it should be built"}, {"name": "write_files", "description": "write a set of files to my project", "parameters": {...}}]
With these two functions, the assistant will have enough agency that it can write a Dockerfile directly to a user’s project. Unfortunately, without best practices, the actual Dockerfile content is never very good.
Here’s an example of the kind of Dockerfile that gets generated when the assistant knows the kind of project but not much else.
# Use the official Node.js image from the Docker HubFROM node:14# Create and change to the app directoryWORKDIR /usr/src/app# Copy the package.json and package-lock.json filesCOPY package*.json ./# Install dependenciesRUN npm install# Copy the rest of the application codeCOPY . .# Expose the port your app runs onEXPOSE 3000# Define the command to run your appCMD ["npm", "start"]
This example shows several standard Dockerfile authoring problems.
- Uses out of date base images.
- Does not employ multi-stage builds.
- Skips newer Docker features such as cache mounts.
So, what can we do about this? Let’s extract some best practices for authoring NPM project Dockerfiles.
Write Dockerfiles for NPM projects using three stages. Do these three steps sequentially.* the first node dependencies stage should be called "deps" and it should fetch the runtime dependencies using npm ci with the --omit=dev flag.* The second Dockerfile stage should be called build and it should be based on the deps stage. It should run npm ci and then npm build* The third Dockerfile stage should select a base image that is recommended by Scout and use that in the FROM line. This stage should do then do three things. 1. it copies the node_modules directory from the deps stage. 2. it copies the dist directory from the build stage. 3. it then runs npm startIf you need to use a RUN statement containing npm ci always add the argument --mount=type=cache,target=/root/.npm to the RUN instruction. The --mount argument should be placed between the word RUN and the npm command.This will cache the npm packages in the docker build cache and speed up the build process.After selecting a base image, get a recommended tag. This recommendation will change over time but start with what is recommended now.
We’ll include this content in any conversation occurring in the context of an NPM project. We’ll also add a third function.
[{"name": "docker_scout_tag_recommendation", "description": "get a recommended tag", "parameters": {...}}]
At the time of writing, here’s the output from our assistant. In this example, the assistant is using the GPT-4 model, but we are seeing the same results with other local models.
# Stage 1 - Downloading dependenciesFROM node:22-slim AS depsWORKDIR /usr/src/appCOPY package*.json ./RUN --mount=type=cache,target=/root/.npm npm ci --omit=dev# Stage 2 - Building applicationFROM deps AS buildRUN --mount=type=cache,target=/root/.npm npm ci && npm build# Stage 3 - Using a recommended base image from ScoutFROM node:22-slimWORKDIR /usr/src/appCOPY --from=deps /usr/src/app/node_modules ./node_modulesCOPY --from=build /usr/src/app/dist ./distCMD [ "npm", "start" ]
This is a big improvement. The three inputs that underlie this improvement are listed below.
- The two top-level instructions that anchor the process our assistant should use:
* First, analyze the project to determine how it should be built. * Once the analysis is complete, create a Dockerfile to build that project.
- The Markdown content that summarizes our Docker specific best practices.
- The functions (
analyze_project, write_files
, anddocker_scout_tag_recommendation
).
Distilling best practices is certainly not easy, but a little can go a long way.Still, it is still hard to know whether this can be considered an improvement over just using code templates. Our continuing investigation centers around whether generative AI will help us to more easily adapt these models to new knowledge, new features, and new project types.
Conversation loop
It’s instructive to go through the actual messages passed between agents here. It’s particularly interesting to look at the interplay between the Assistant and the Tool agents. Near the end of the exchange, there is an unexpected twist where the write_files
tool complains that it was passed an invalid JSON file. In this case, the assistant actually did generate invalid JSON and the tool was right to complain. However, the assistant also responded to this error by fixing the JSON and calling the tool a second time! That was unscripted.
Another interesting example of an unscripted moment is the last message from the assistant. The assistant correctly determines that the task is now complete and generates a summary of the work that was done and why.
Transcript
System:
You are an assistant who specializes in authoring Dockerfiles for projects. Since you are an expert and know about their project, be definitive about recommendations.
User:
- First, analyze the project to determine how it should be built.
- Once the analysis is complete, create a Dockerfile to build that project.
Assistant:
[{:function {:arguments "{}" :name "analyze_project"}}]
Tool (analyze_project
):
This is an NPM project.
System:
Write Dockerfiles for NPM projects using three stages. Do these three steps sequentially …
…
… (system prompts excluded for brevity) ..
…
Assistant:
[{:function {:arguments "{ \"repository\": \"node\"}" :name "docker_scout_tag_recommendation"}}]
Tool (docker_scout_tag_recommendation
):
22-slim
Assistant:
[{:function {:arguments "{ \"files\": [ { \"path\": \"Dockerfile\", \"content\": `# Stage 1 - the build processFROM node:22-slim AS depsWORKDIR /usr/src/appCOPY package*.json ./RUN --mount=type=cache,target=/root/.npm npm ci --omit=dev# Stage 2 - the production environmentFROM deps AS buildRUN npm ci && npm run build# Stage 3 - the production environmentFROM node:22-slimWORKDIR /usr/src/appCOPY --from=deps /usr/src/app/node_modules ./node_modulesCOPY --from=build /usr/src/app/dist ./distCMD [ \"npm\", \"start\" ]`, \"executable\": false } ]}" :name "write_files"} :id "call_6VVCLiXcIBNyEGPQwftR7xEV"}]
Tool:
function call write_files
failed call exited with non-zero code (1): call exited with non-zero code (1): #error {\r\n :cause Unexpected character (‘`’)
Assistant:
I apologize for the error. Let me try that again.
[{:function {:arguments "{ \"files\": [ { \"path\": \"Dockerfile\", \"content\": \"FROM node:22-slim AS deps\\nWORKDIR /usr/src/app\\nCOPY package*.json ./\\nRUN --mount=type=cache,target=/root/.npm npm ci --omit=dev\\n\\nFROM deps AS build\\nRUN npm ci && npm run build\\n\\nFROM node:22-slim\\nWORKDIR /usr/src/app\\nCOPY --from=deps /usr/src/app/node_modules ./node_modules\\nCOPY --from=build /usr/src/app/dist ./dist\\nCMD [ \\\"npm\\\", \\\"start\\\" ]\", \"executable\": false } ]}" :name "write_files"}}]
Tool (write_files
):
wrote 1 files: Dockerfile
Assistant:
I have written the Dockerfile for your NPM project. You can find it in your project folder. This Dockerfile uses a multi-stage build process to efficiently build and run your application within a Docker container. The first stage fetches the runtime dependencies, the second stage builds the application, and the final stage runs the application. Please let me know if you need further assistance.
Summary
For a generative AI assistant to improve its ability to generate Dockerfile content, we have identified both tools and stack-specific best practice content as key enablers. Incorporating these elements into a generative AI based assistant can serve as an alternative to code templates. We continue to evaluate whether this approach allows us to more easily adapt to new project types.
As always, feel free to follow along in our public repo and reach out. Everything we’ve discussed in this blog post is available for you to try on your own projects.
For more on what we’re doing at Docker, subscribe to our newsletter.